The ensemble Kalman filter (EnKF) and its versatile variants are renowned for their remarkable data assimilation capabilities in diverse domains such as atmospheric, oceanic, hydrologic, biomedical, biologic, and petroleum reservoir systems. By providing a platform for thought-provoking presentations and meaningful discussions, the EnKF workshop seeks to foster collaboration among technical experts, practitioners, researchers, and students. Together, we will showcase cutting-edge research findings, exchange practical insights, and collectively explore uncharted territories by identifying crucial challenges. Join us to deepen your knowledge, expand your network, and contribute to the advancement of data assimilation.
Venue
- Solstrand Hotel, Os, Norway.
- June 17 - 19, 2024.
- The local climatology
- Physical attendance only (no virtual participation or videoconferencing).
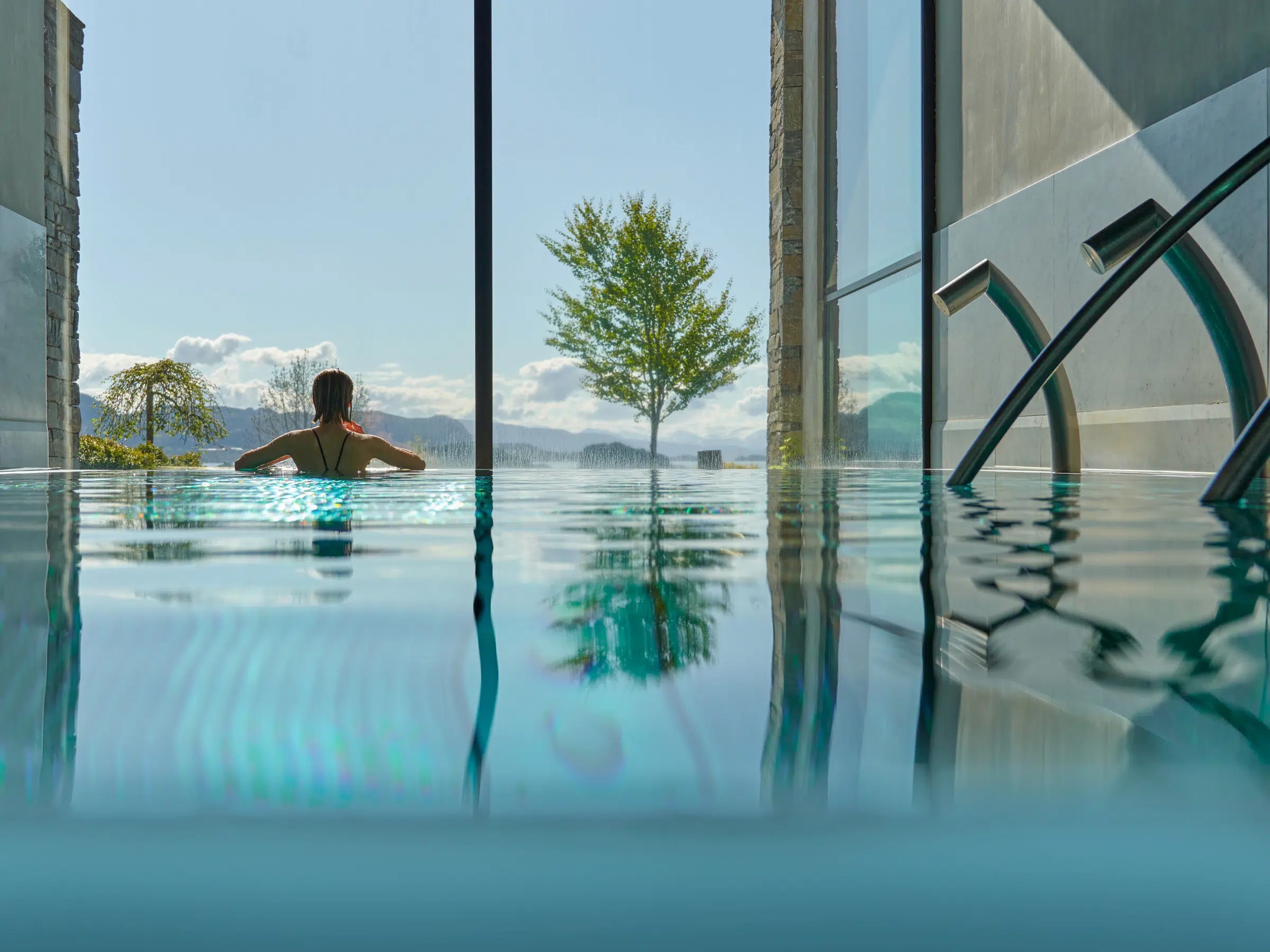
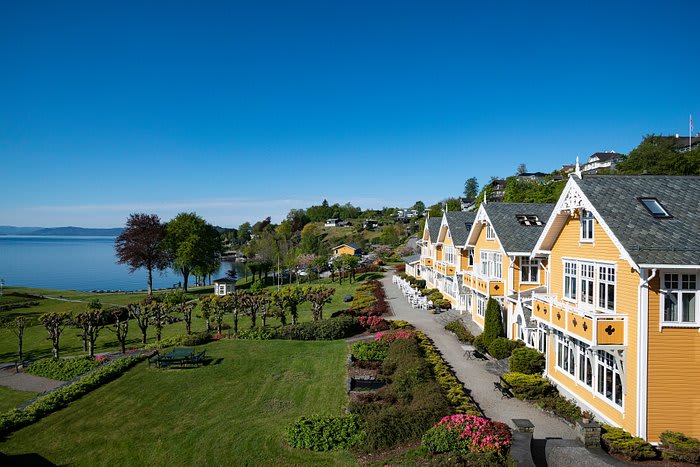
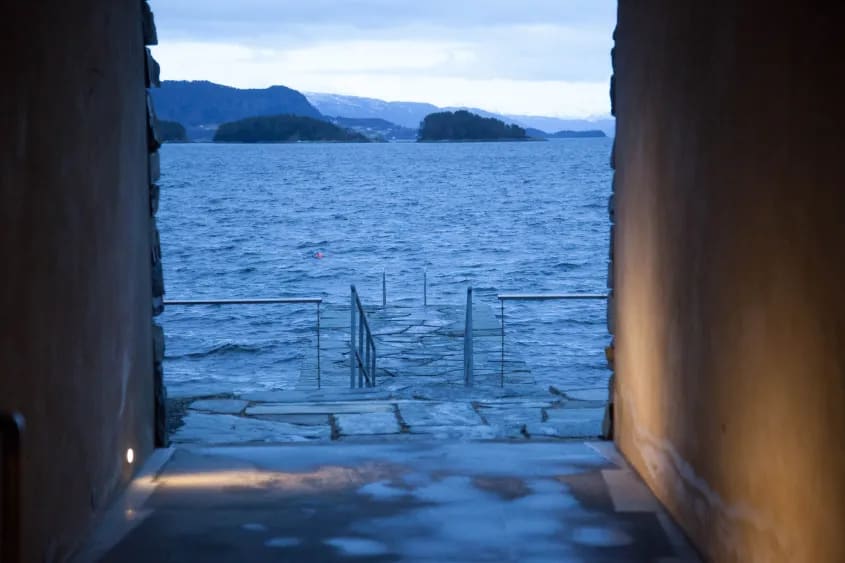
Program
- Participants must arrange their own travel to/from Bergen.
- A chartered bus will take us from downtown Bergen to Solstrand hotel.
- The unsession will consist of an open discussion on benchmarking. The sharing and adaption of open benchmark models and datasets is one of the fuels powering the rapid progress of ML and AI. The DA community has a handful of well-known benchmark models, but implementations vary and comparisons are not always straightforward. On the data side, there is much scope for improvement. In this guided discussion, we will ask, “What do we have?”, “What do we need?”, and “How will we get there?”. We will not solve the issue, but we will emerge with a better understanding of what needs to be done, and who is up for doing it!
- There are some moderate hiking opportunities in the area.
- The hotel includes pools with spa facilities, with access to the fjord for more daring swimmers.
Invited speakers
-
Alban Farchi
Reseacher, CEREA, École des Ponts ParisTech, France
Online model error correction with neural networks – from theory to the ECMWF forecasting system -
Tijana Janjic
Professor, KU Eichstätt Ingolstadt, Germany
Learning model parameters from observations by combining data assimilation and machine learning -
Chris Snyder
Researcher, NSF NCAR, USA
Sampling error in the ensemble Kalman filter for small ensembles and high-dimensional states -
Julien Brajard
Researcher, NERSC, Norway
The interplay between data assimilation and artificial intelligence -
Berent Å. S. Lunde
Researcher, Equinor, Norway
Linear triangular transport at scale
Presentations
Dean S. Oliver | talk | Importance Weighting in Hybrid Iterative Ensemble Smoothers for Data Assimilation |
Berent Å. S. Lunde | talk | Linear triangular transport at scale |
Xiaohui Wang | talk | Improving coastal flooding forecasts with data assimilation using crowdsourced observations |
Matthias Morzfeld | talk | Noise informed covariance estimation |
Chris Snyder | talk | Sampling error in the ensemble Kalman filter for small ensembles and high-dimensional states |
Marco Bajo | talk | Modelling the sea level in the Mediterranean Sea with an Ensemble Kalman Filter |
Simone Spada | talk | Ensemble Kalman Filter Strategies for Efficient Data Assimilation in Geosciences |
Xiaodong Luo | talk | Cross-validation in an iterative ensemble smoother: Stopping earlier for better |
Yan Chen | talk | A Global Ocean Assimilation System using the localized weighted ensemble Kalman filter |
Tijana Janjic | talk | Learning model parameters from observations by combining data assimilation and machine learning |
Eliott Lumet | talk | Reduced-cost EnKF for parameter estimation of microscale atmospheric pollutant dispersion models |
Luxi Yu | talk | Online State and Dynamic Parameter Estimation in Biotherapeutic Production through Ensemble Kalman Filtering |
Ian Grooms | talk | An ensemble adjustment Kalman filter with model-space localization |
Alban Farchi | talk | Online model error correction with neural networks -- from theory to the ECMWF forecasting system |
Yiguo Wang | talk | Post-processing climate reanalysis with the ensemble Kalman smoother |
Mélanie Rochoux | talk | Challenges in building a system for assimilating airborne thermal infrared data to predict wildland fire behavior |
Jeffrey van der Voort | talk | A Multi-Fidelity Ensemble Kalman Filter with a machine learned surrogate model |
Yue (Michael) Ying | talk | Introducing NEDAS: the Next-generation Ensemble Data Assimilation System |
Naratip Santitissadeekorn | talk | Ensemble-based method for Hawkes-process network construction from time-series of count data |
Julien Brajard | talk | The interplay between data assimilation and artificial intelligence |
Patrick N. Raanes | talk | Ensemble control algorithms |
Martin Verlaan | poster | Hybrid physics-AI-model applied to estuarine hydrodynamics |
Femke Vossepoel | poster | Localisation in iterative ensemble smoothers for coupled nonlinear multiscale models |
Jenny Soonthornrangsan | poster | ESMDA for improving land subsidence prediction from a data-driven and physics-based modeling approach: An application to Bangkok, Thailand |
Luisa d'Amore | poster | Space-Time Decomposition of Kalman Filter |
Gaël Descombes | poster | Satellite air quality data assimilation using the Chimere-DART EAKF for the CAMs EvOlution project |
Maryam Ramezani Ziarani | poster | Towards the assimilation of dual-polarization radar data |
Heng Xiao | poster | Ensemble Neural Filtering: Overcoming Small-Ensemble Limitations in Data Assimilation |
Luxi Yu | poster | Real-time Optimization and Control of Biotherapeutic Product Quality through EnKF and Machine Learning with EnOpt |
Matthias Morzfeld | poster | A theory for why even simple covariance localization is so useful in ensemble Kalman filtering |
Laurent Bertino | poster | Reconstruction of Arctic sea ice thickness (1991-2010) based on hybrid machine learning and data assimilation. |
Rolf J. Lorentzen | poster | Data assimilation featuring deep learning of petrophysical model errors |
Kristian Fossum | poster | Improved subsurface uncertainty quantification with multi-fidelity scenario evaluation |
Mathias Methlie Nilsen | poster | Robust Wind-powered Well Control Optimization |
Sergey Alyaev | poster | AI-based multi-modal interpretation and extrapolation of geophysical logs |
Photos
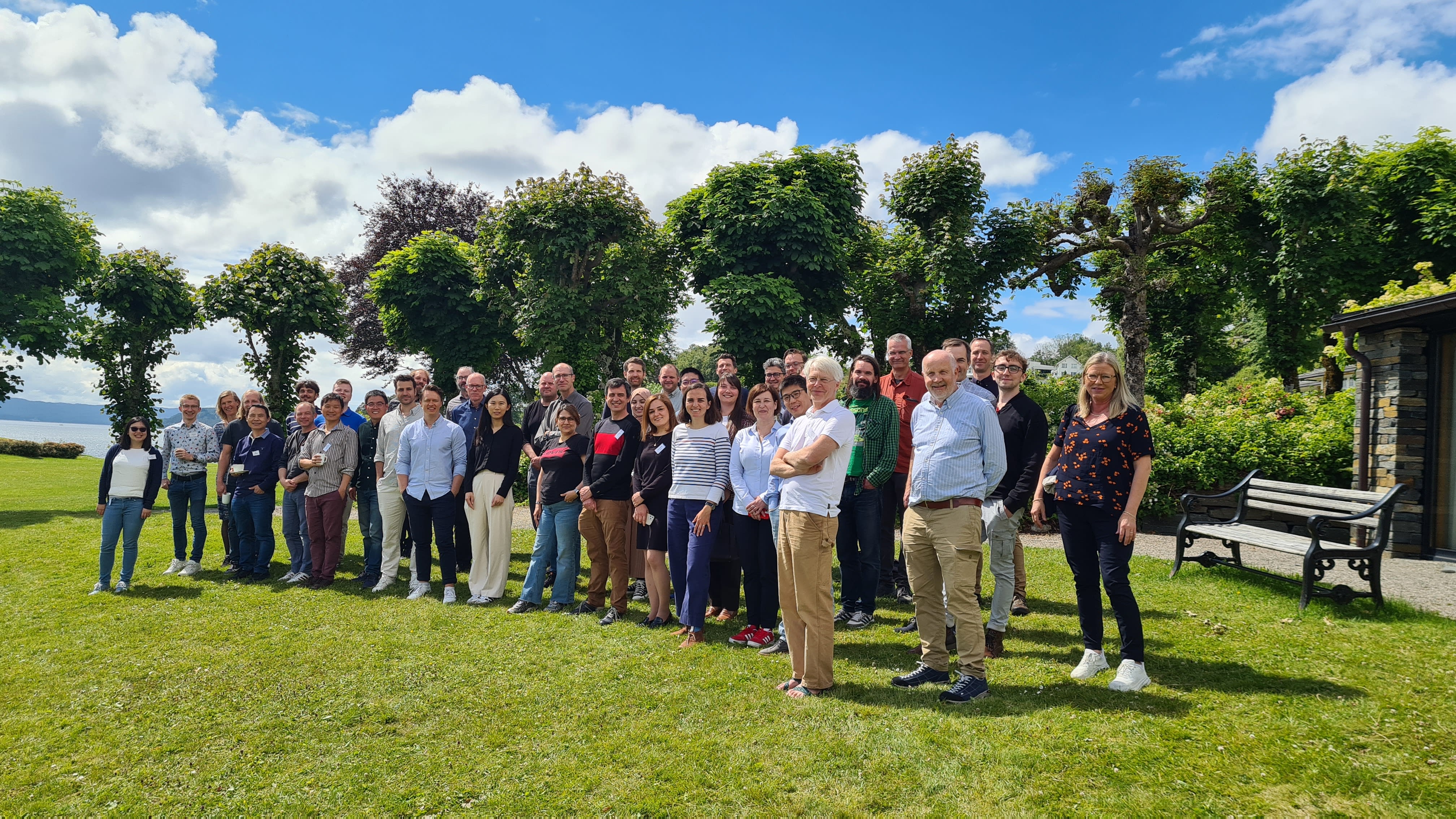
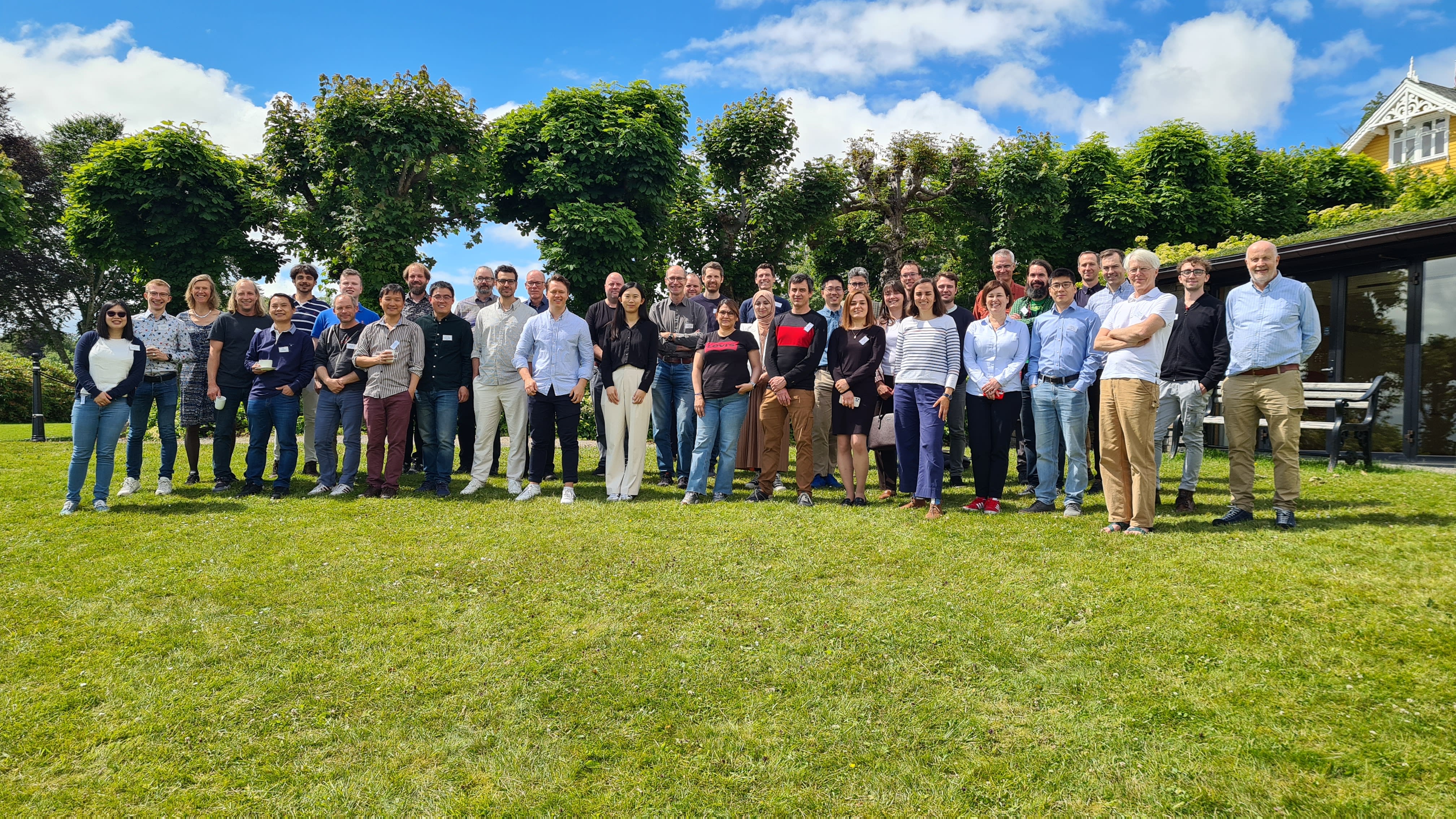
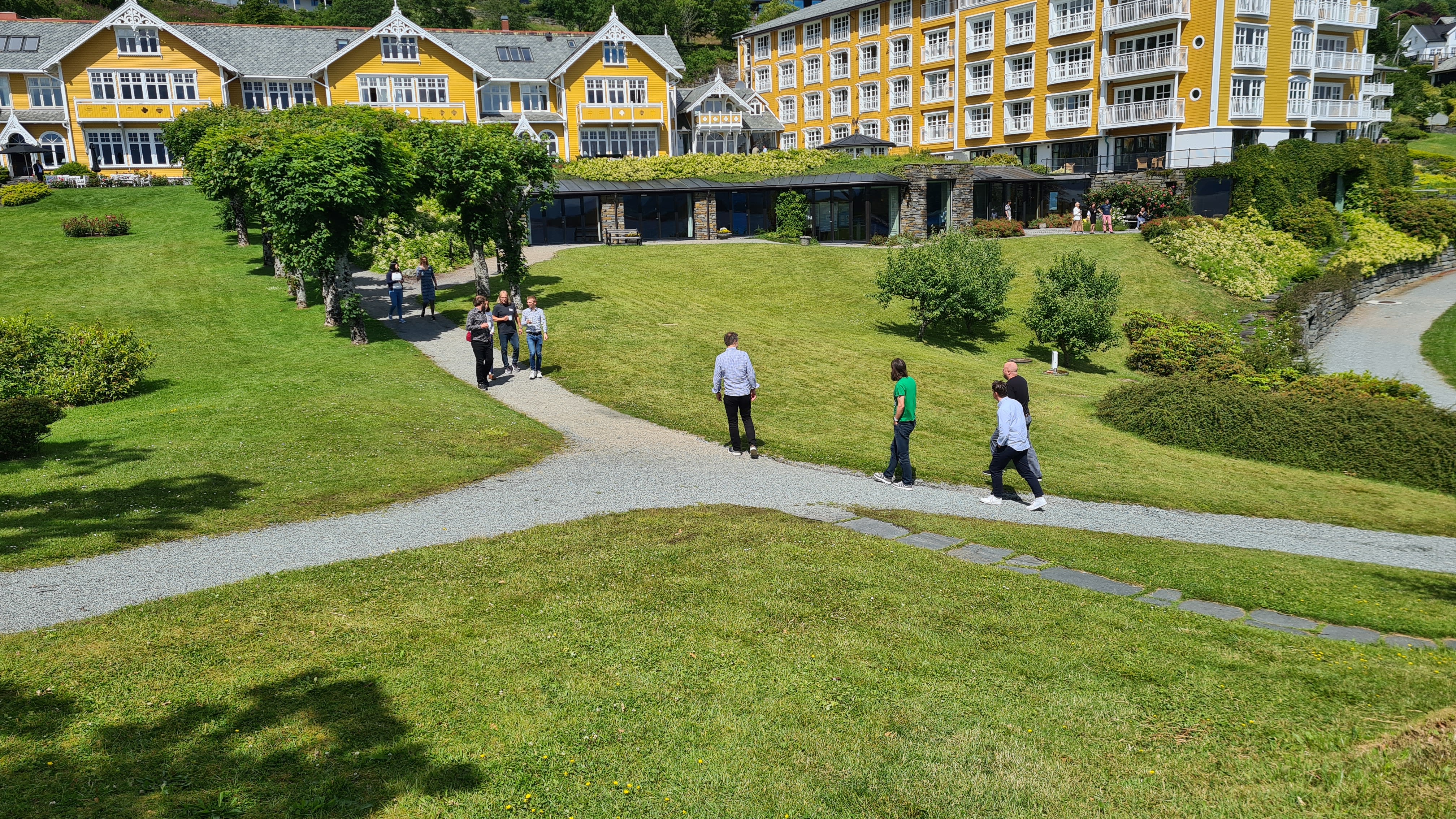
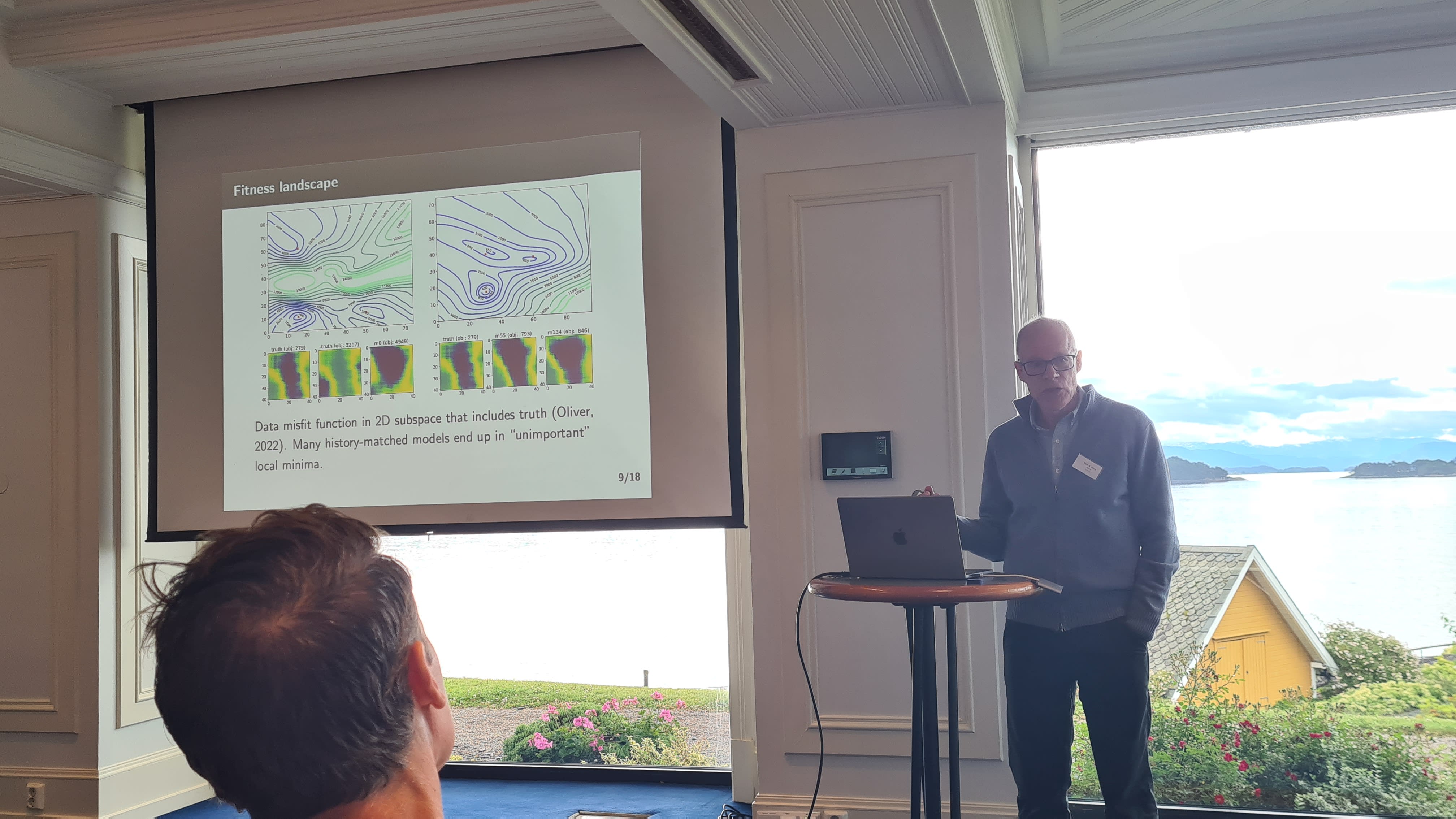
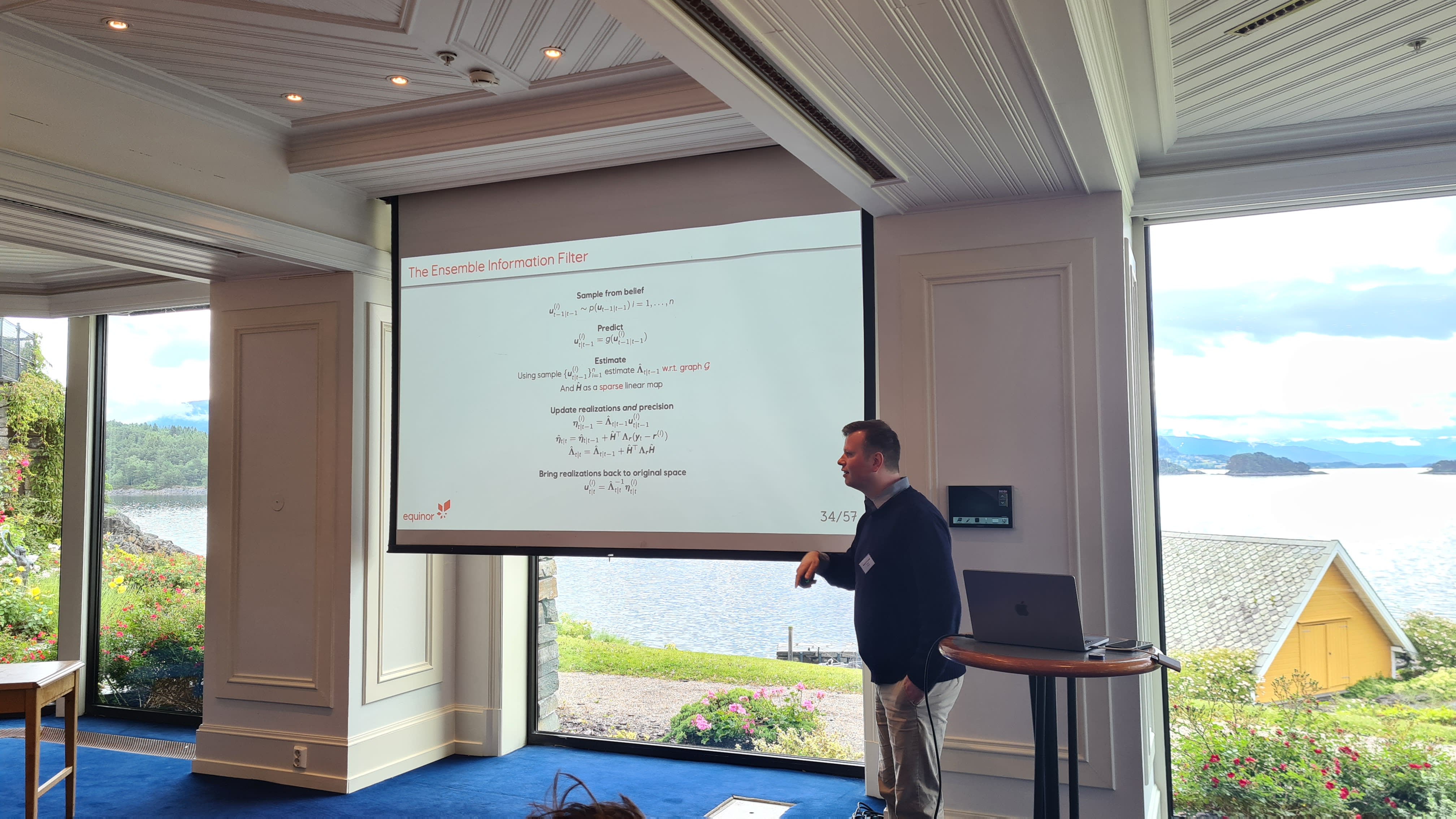
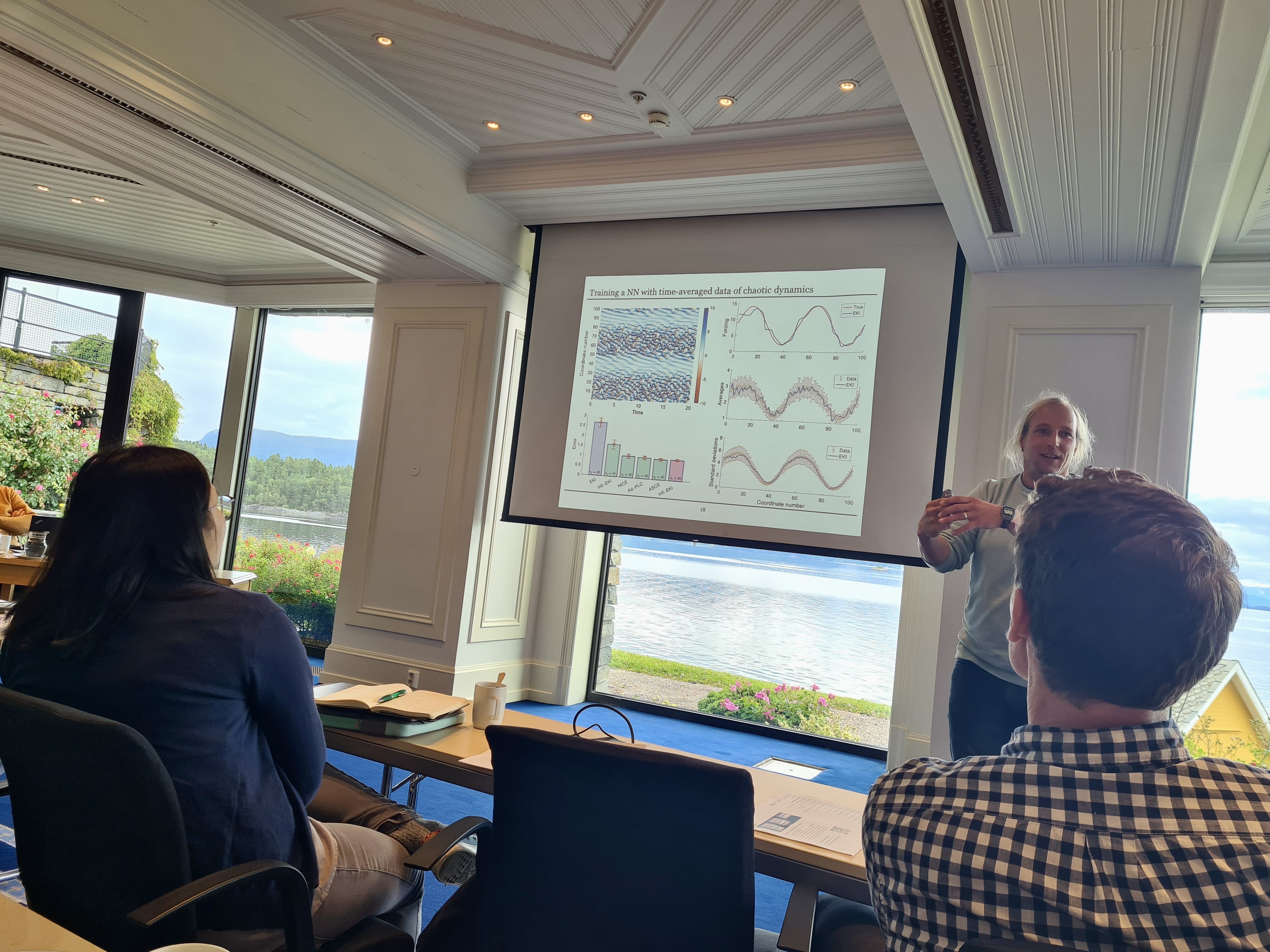
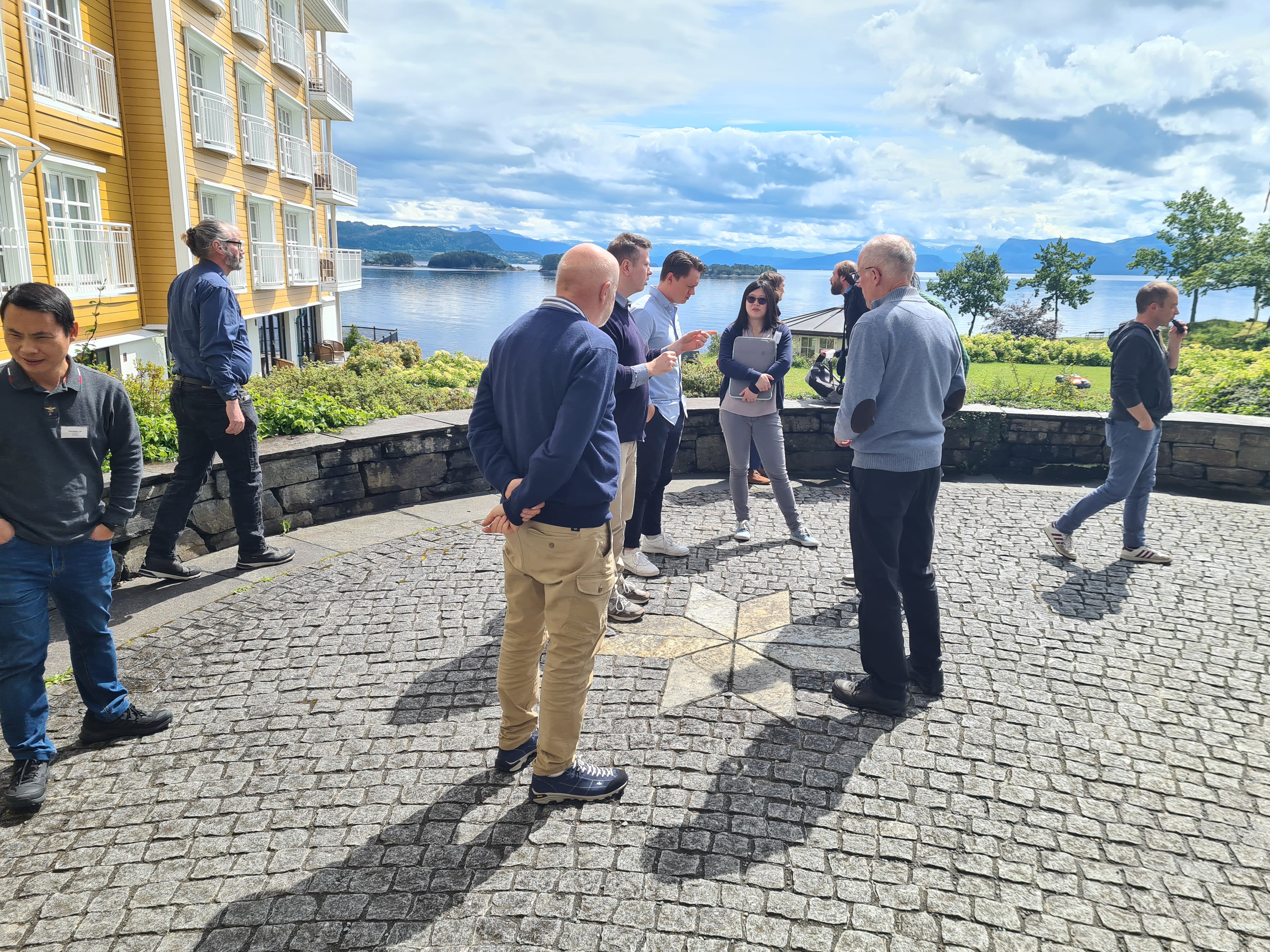
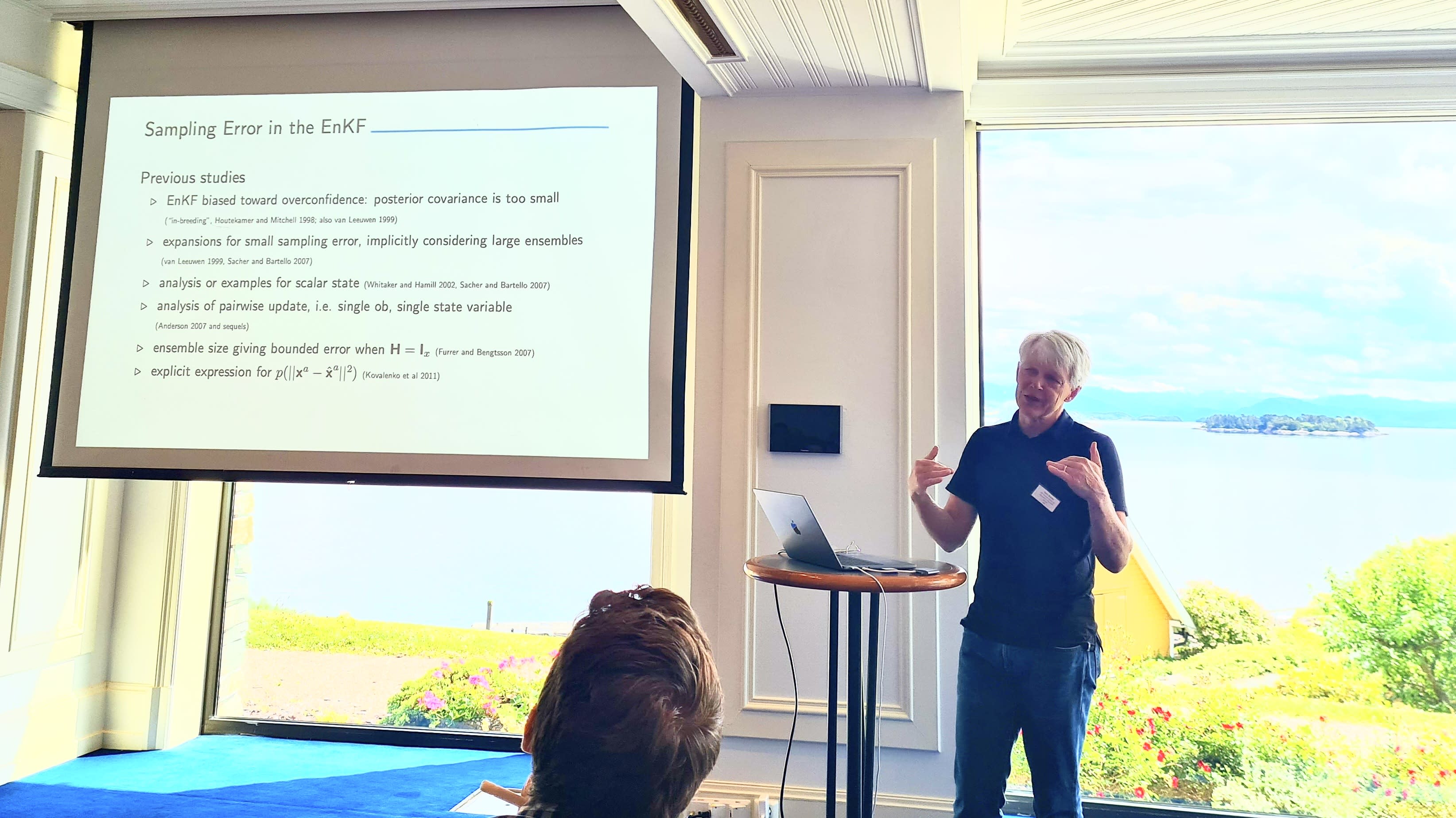
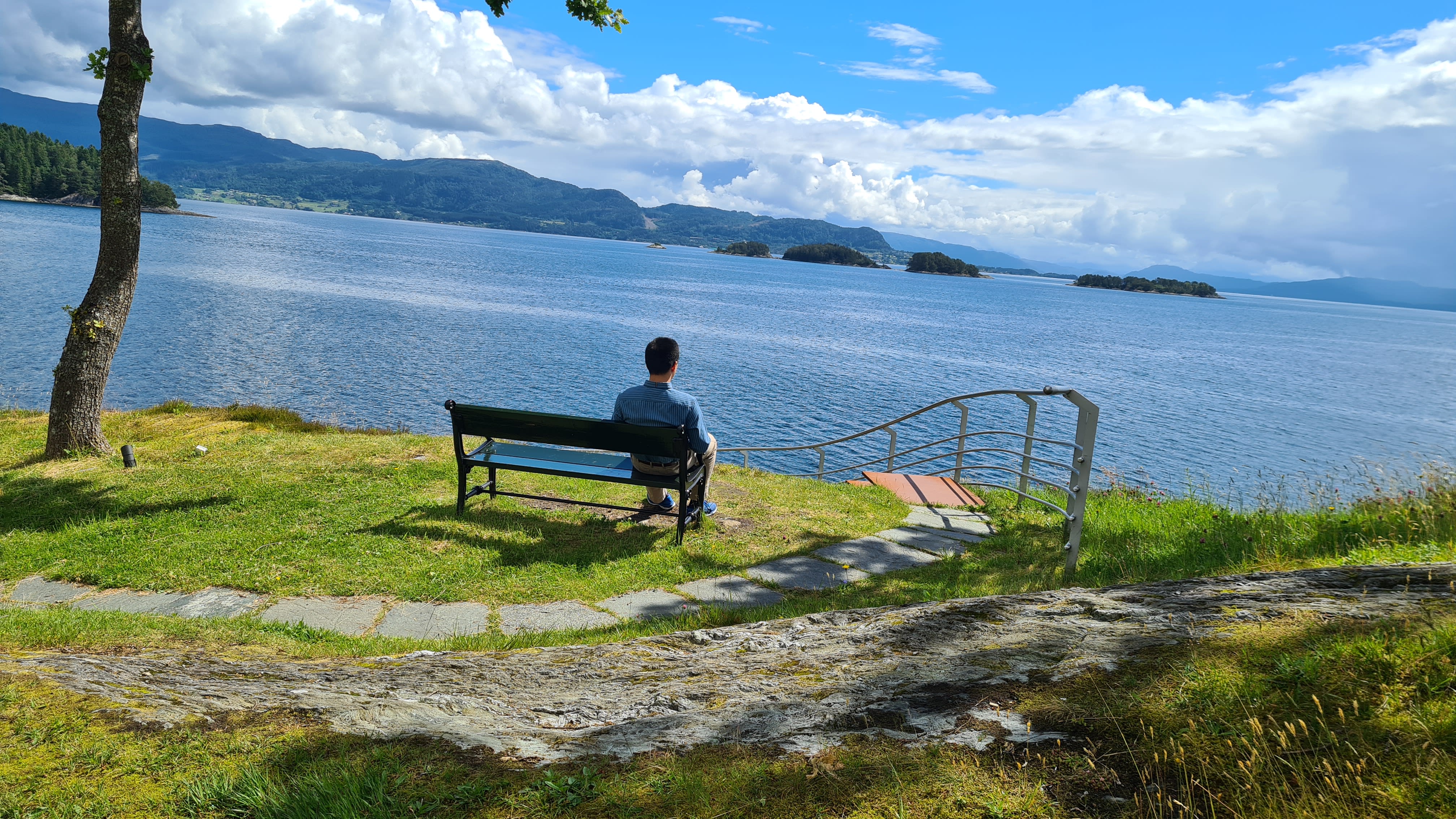
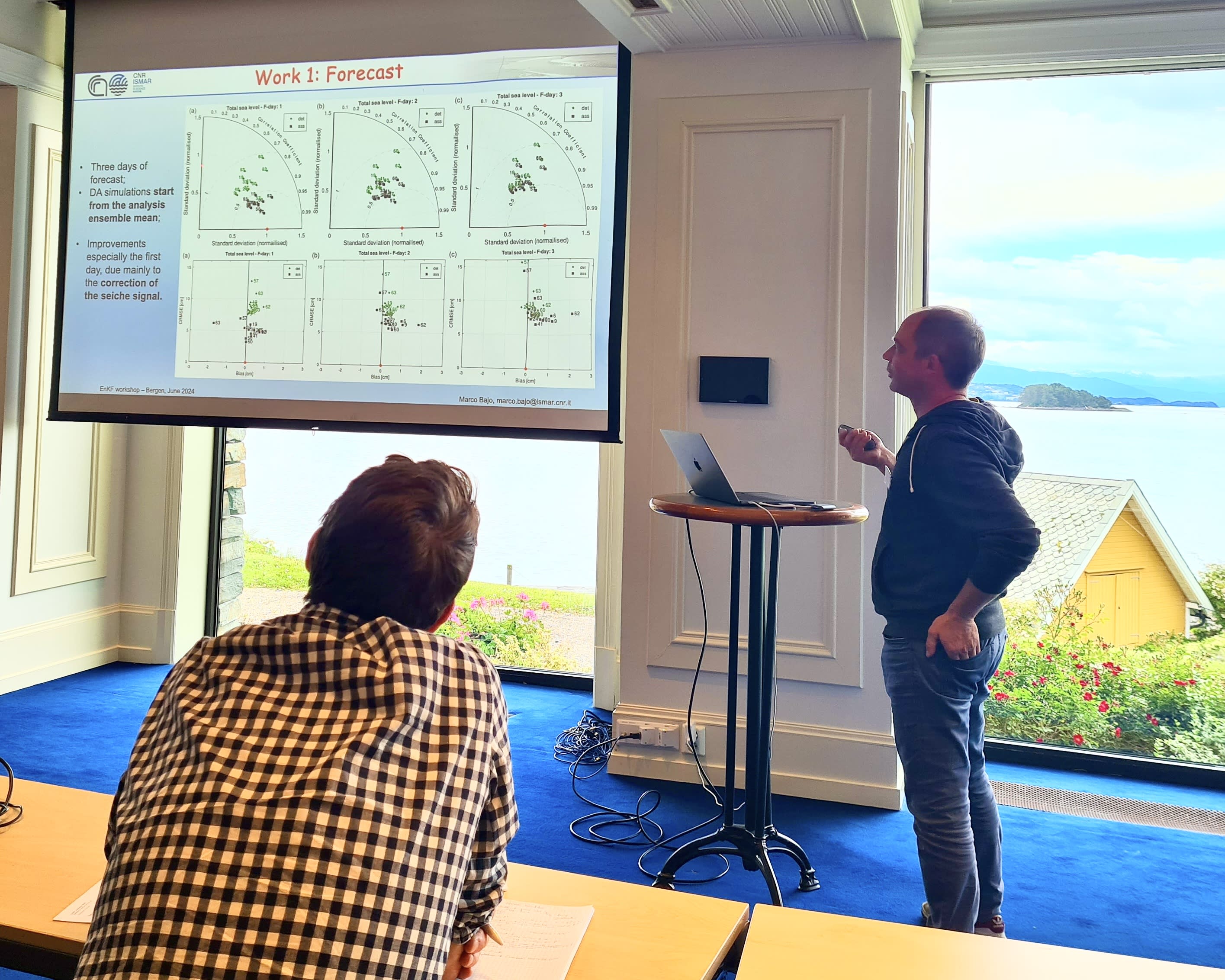
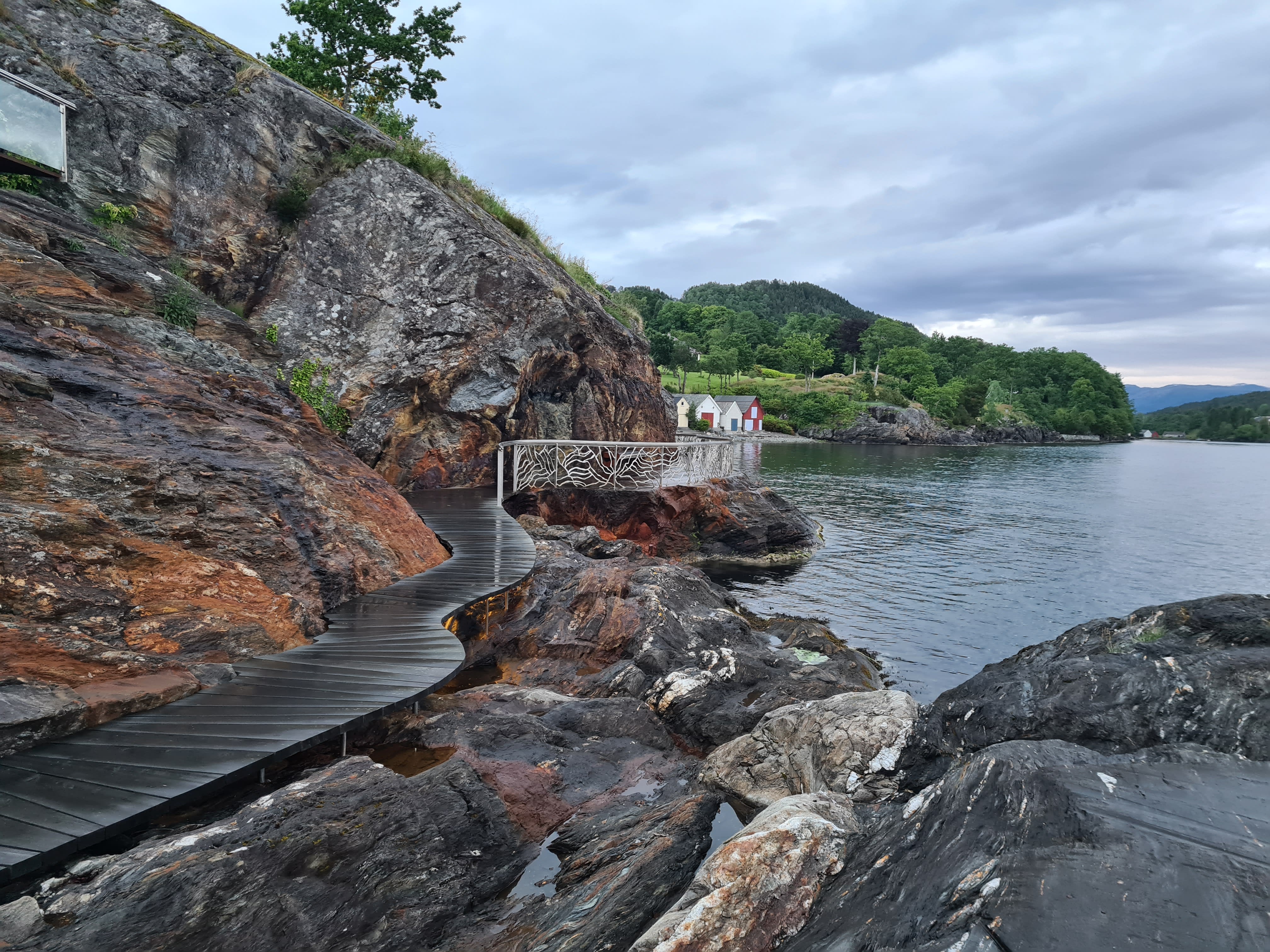
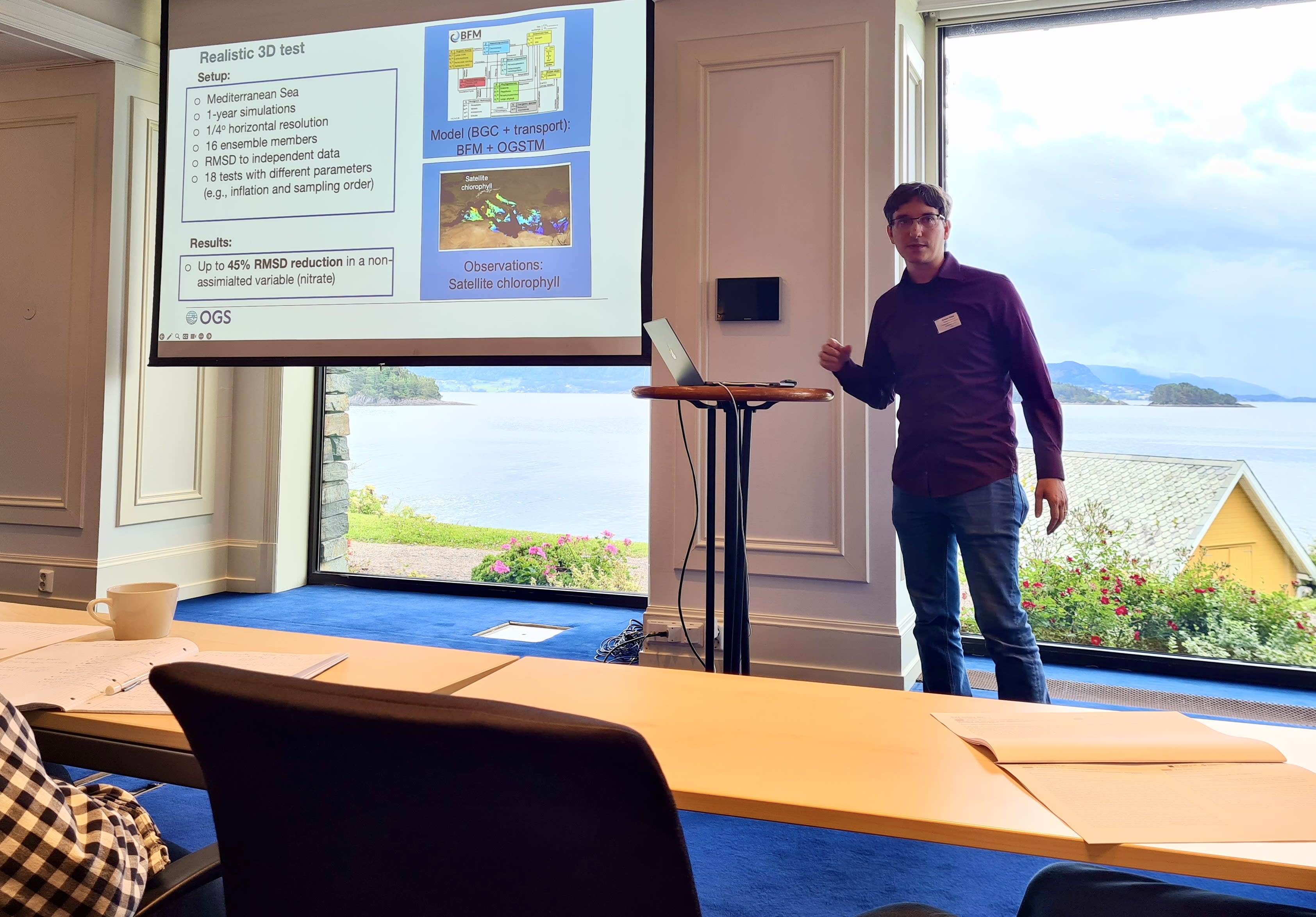
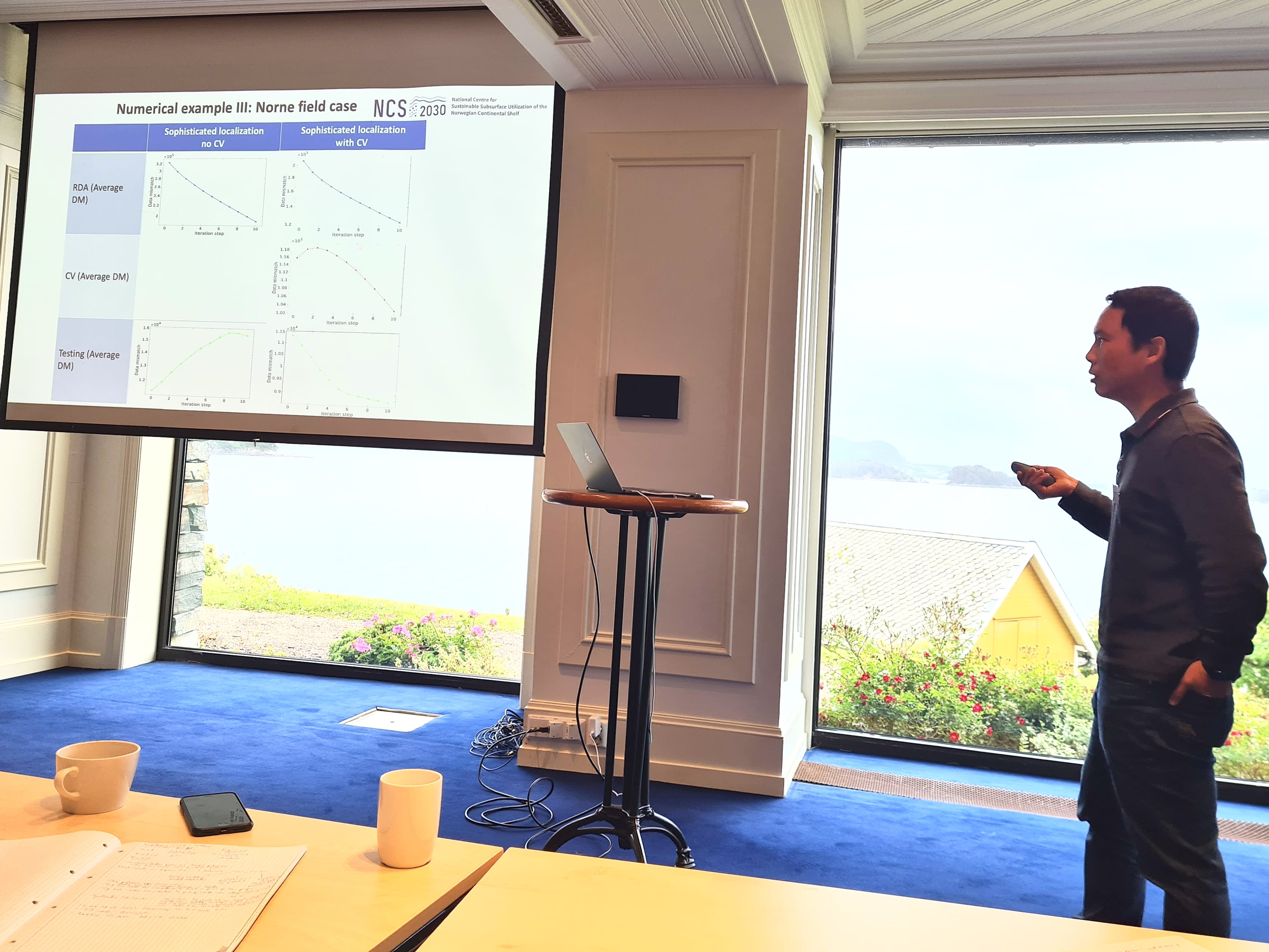
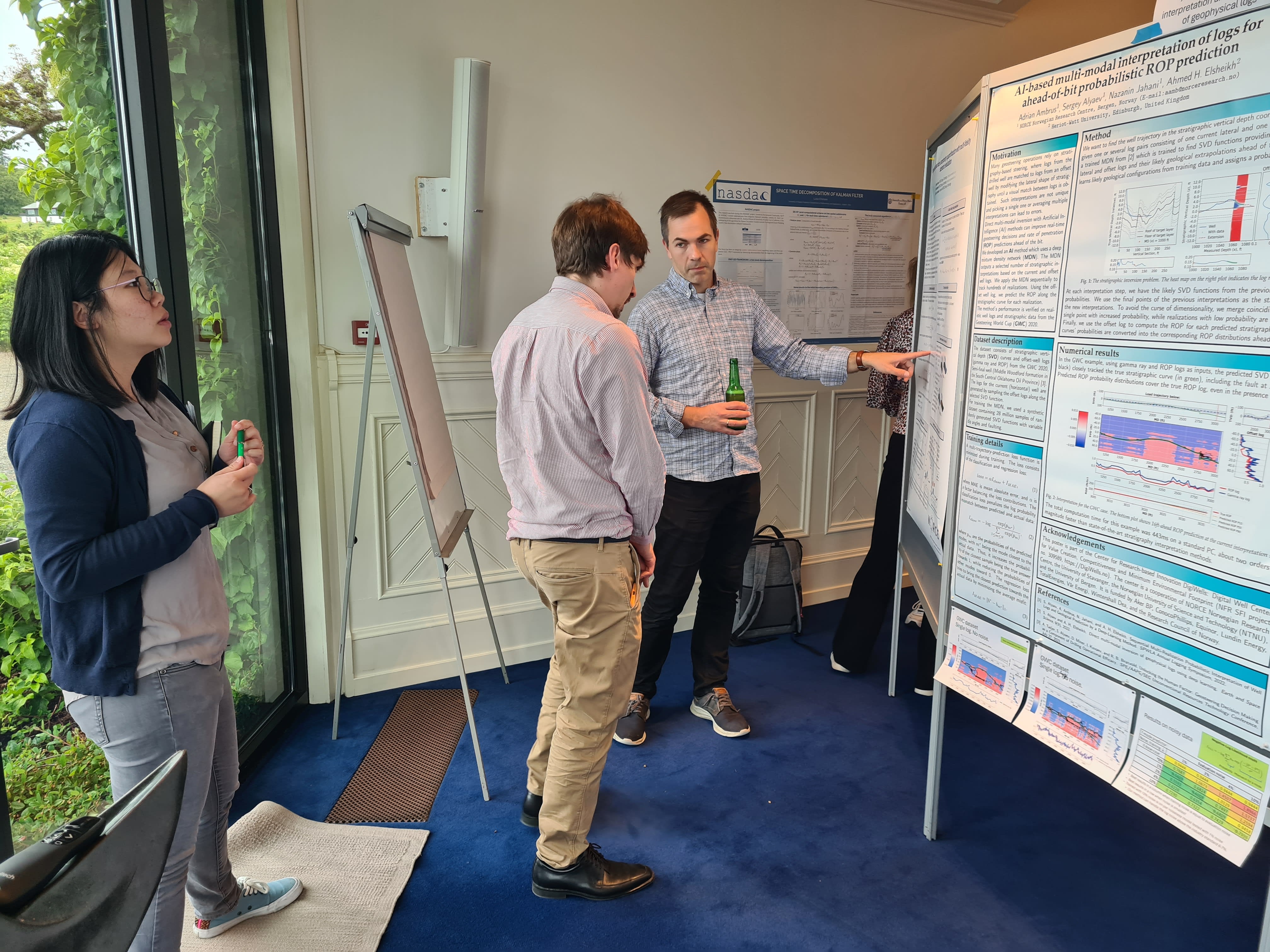
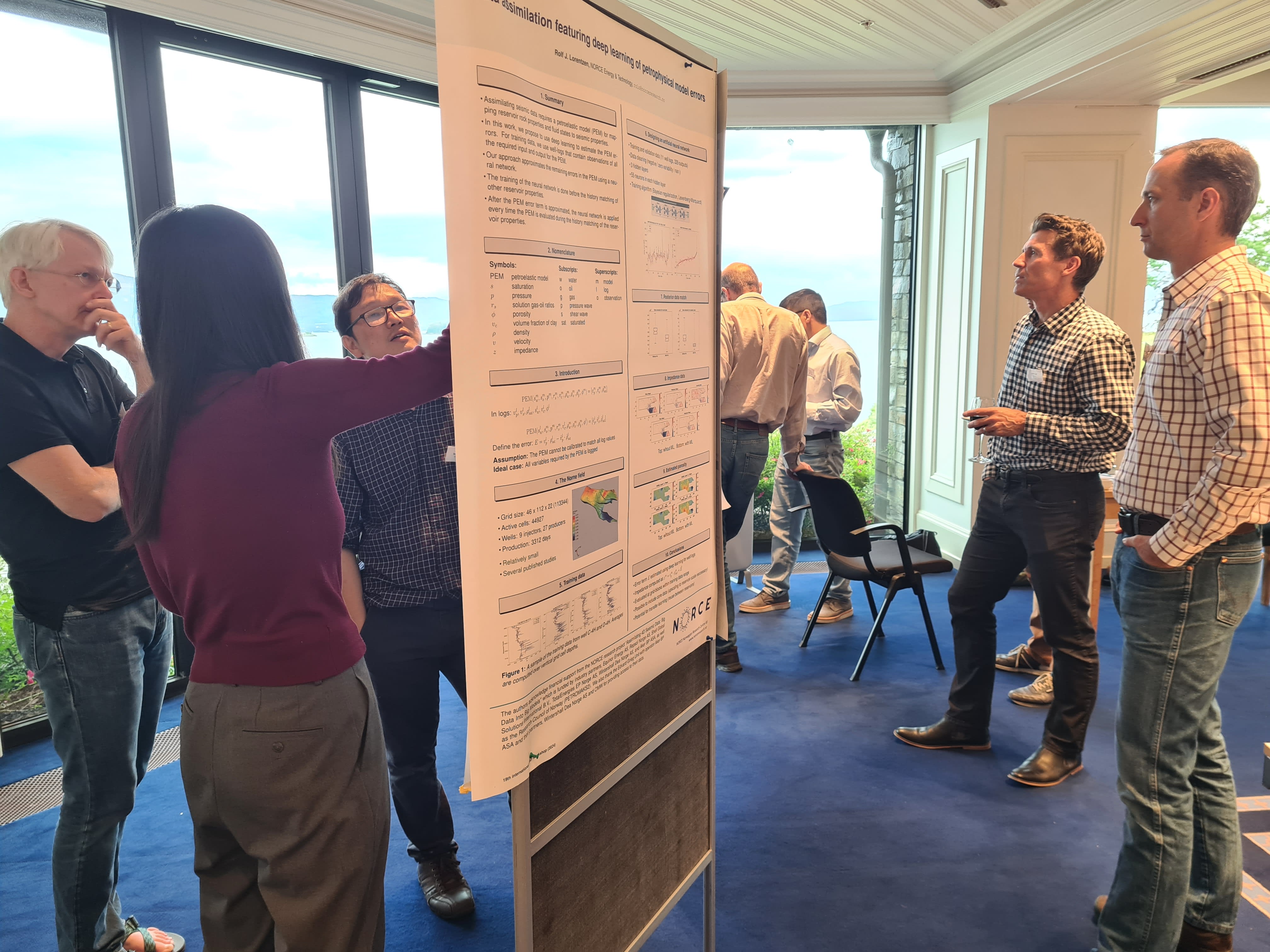
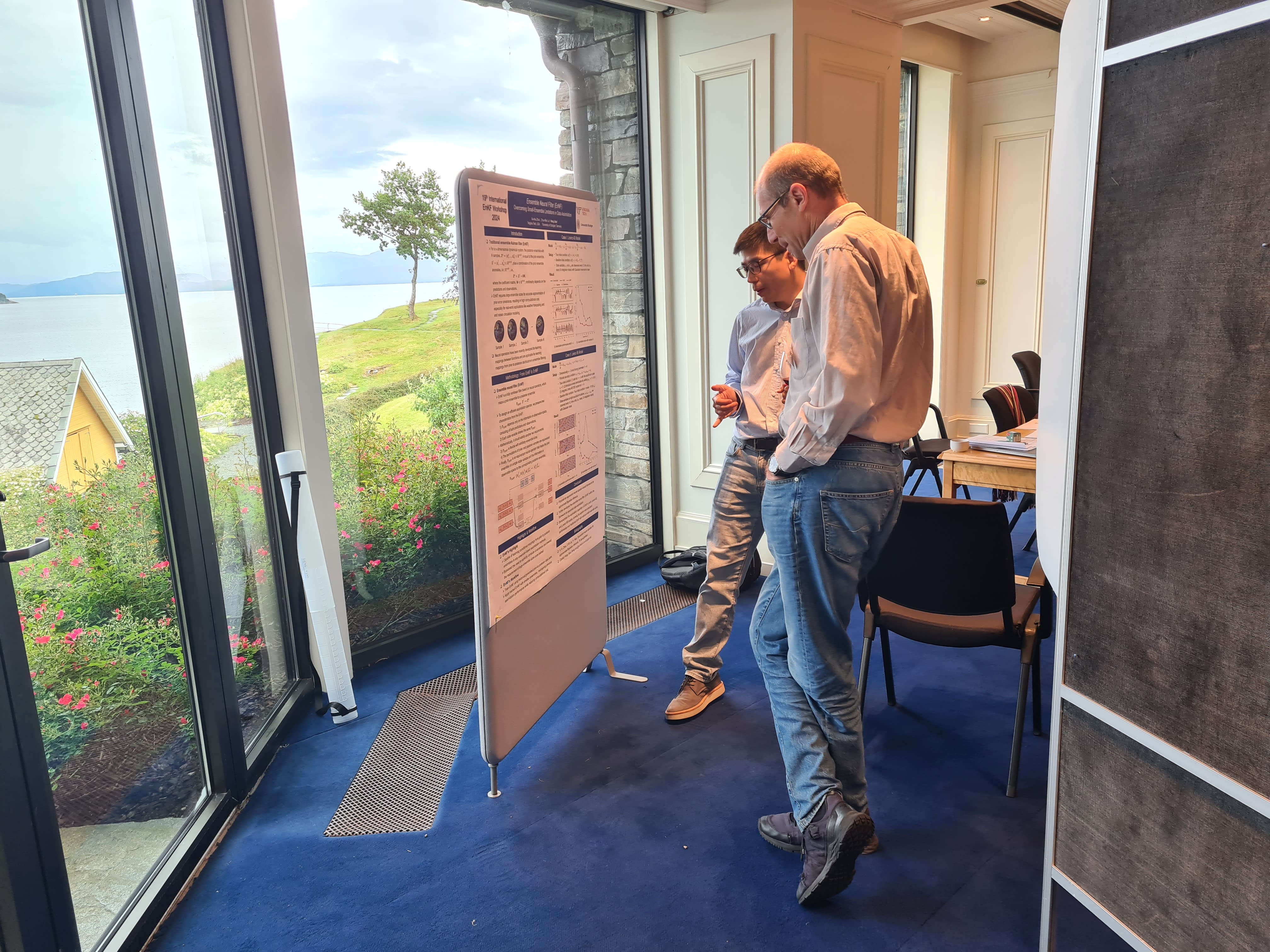
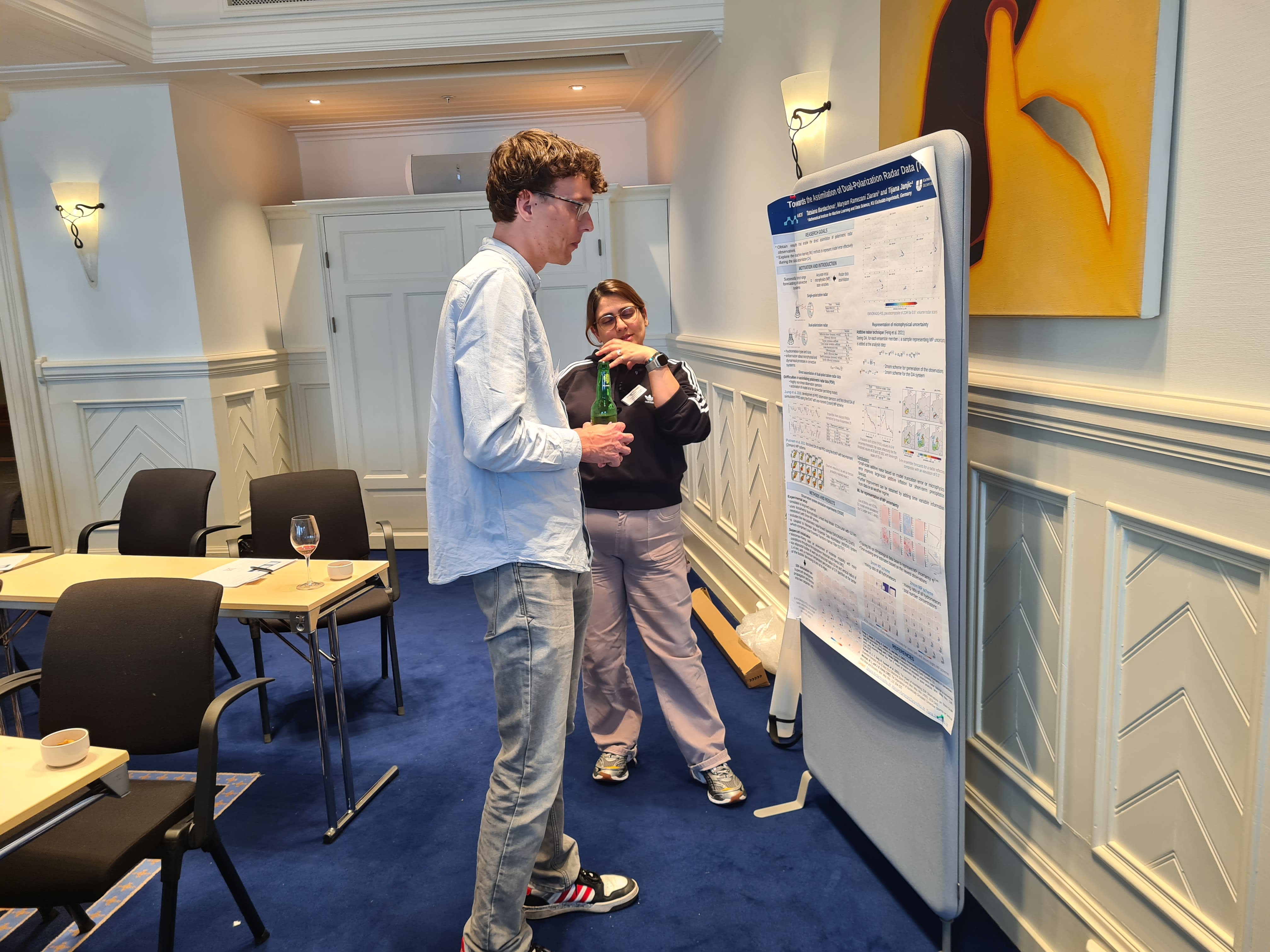
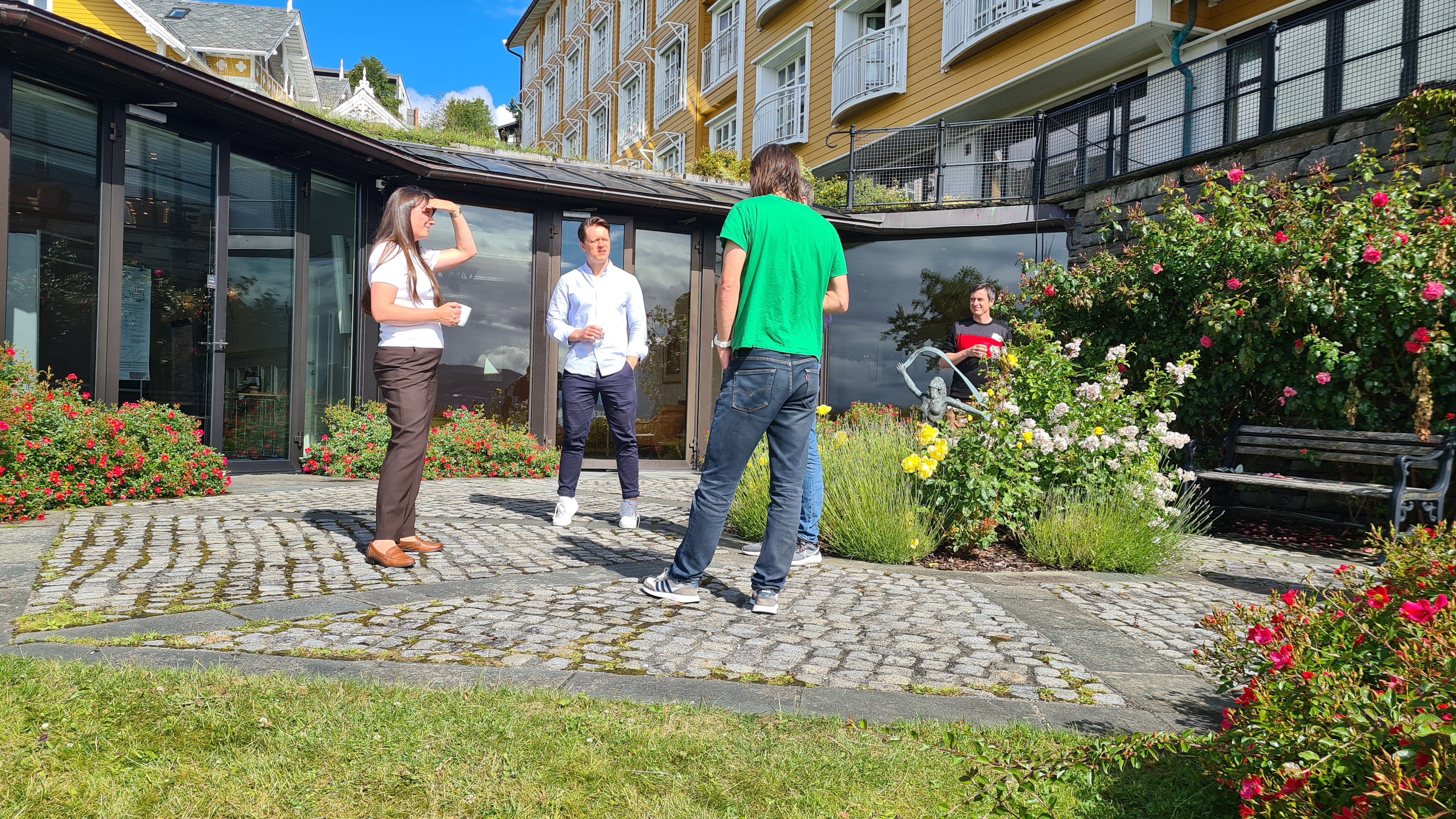
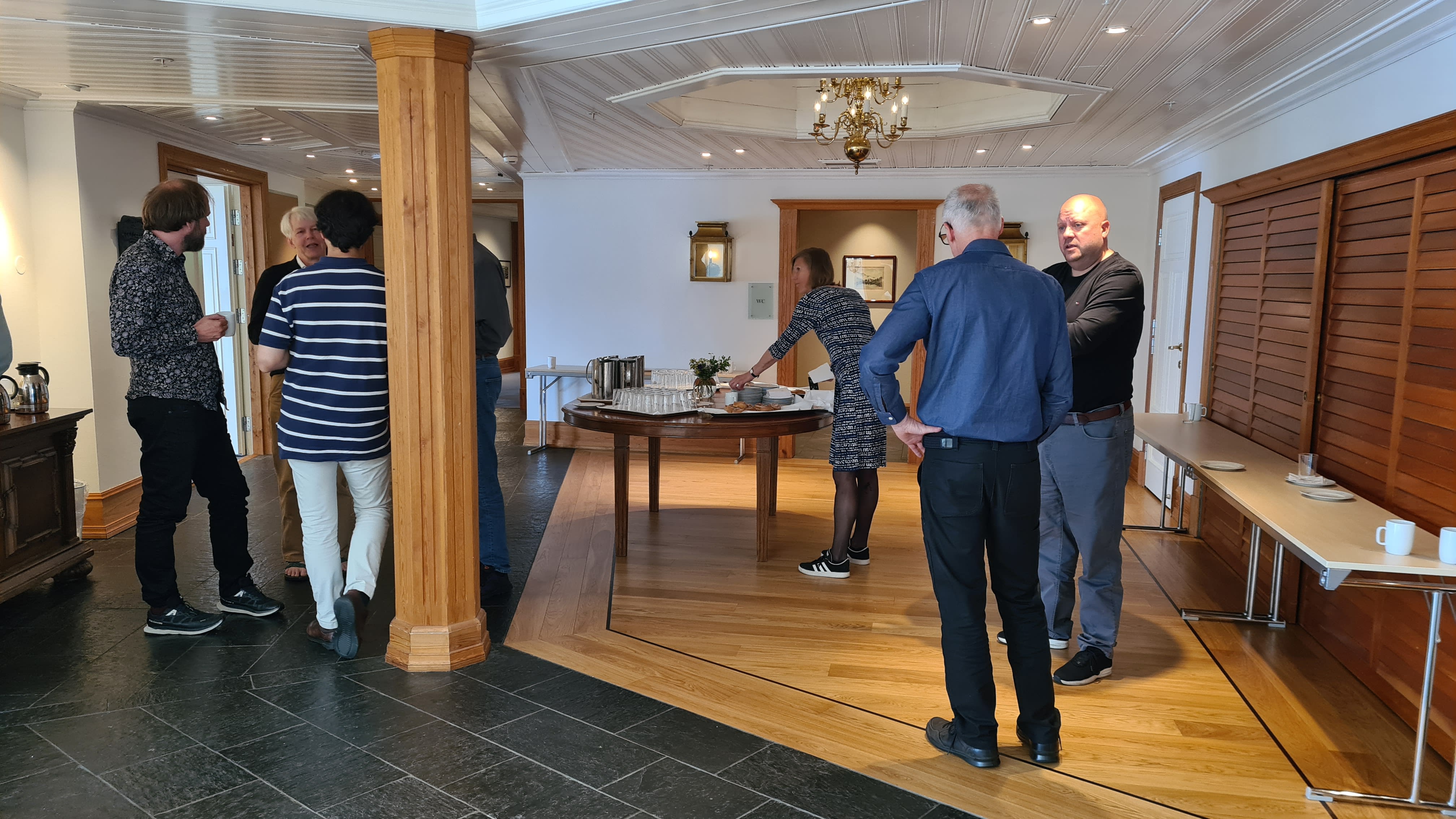
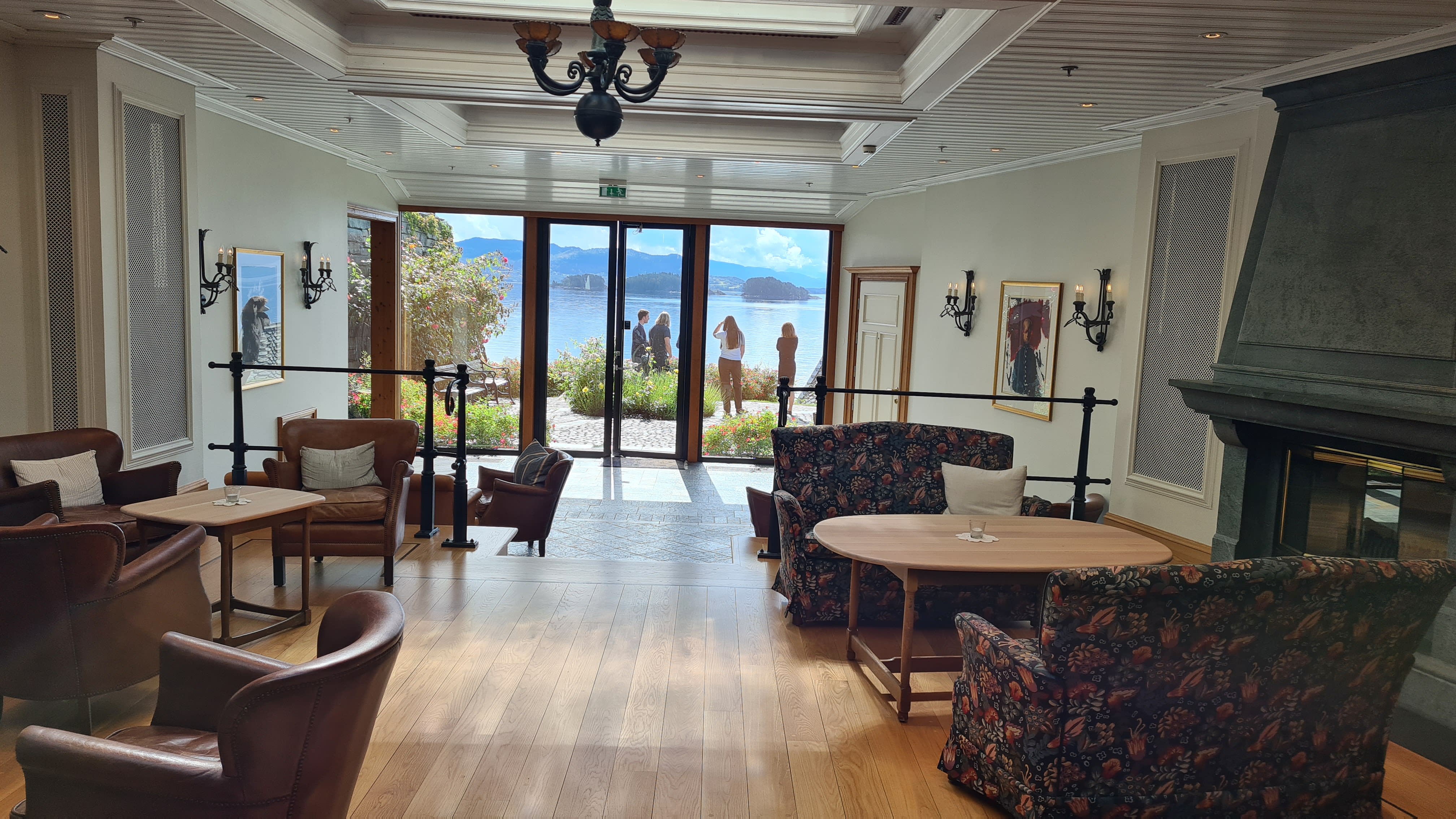
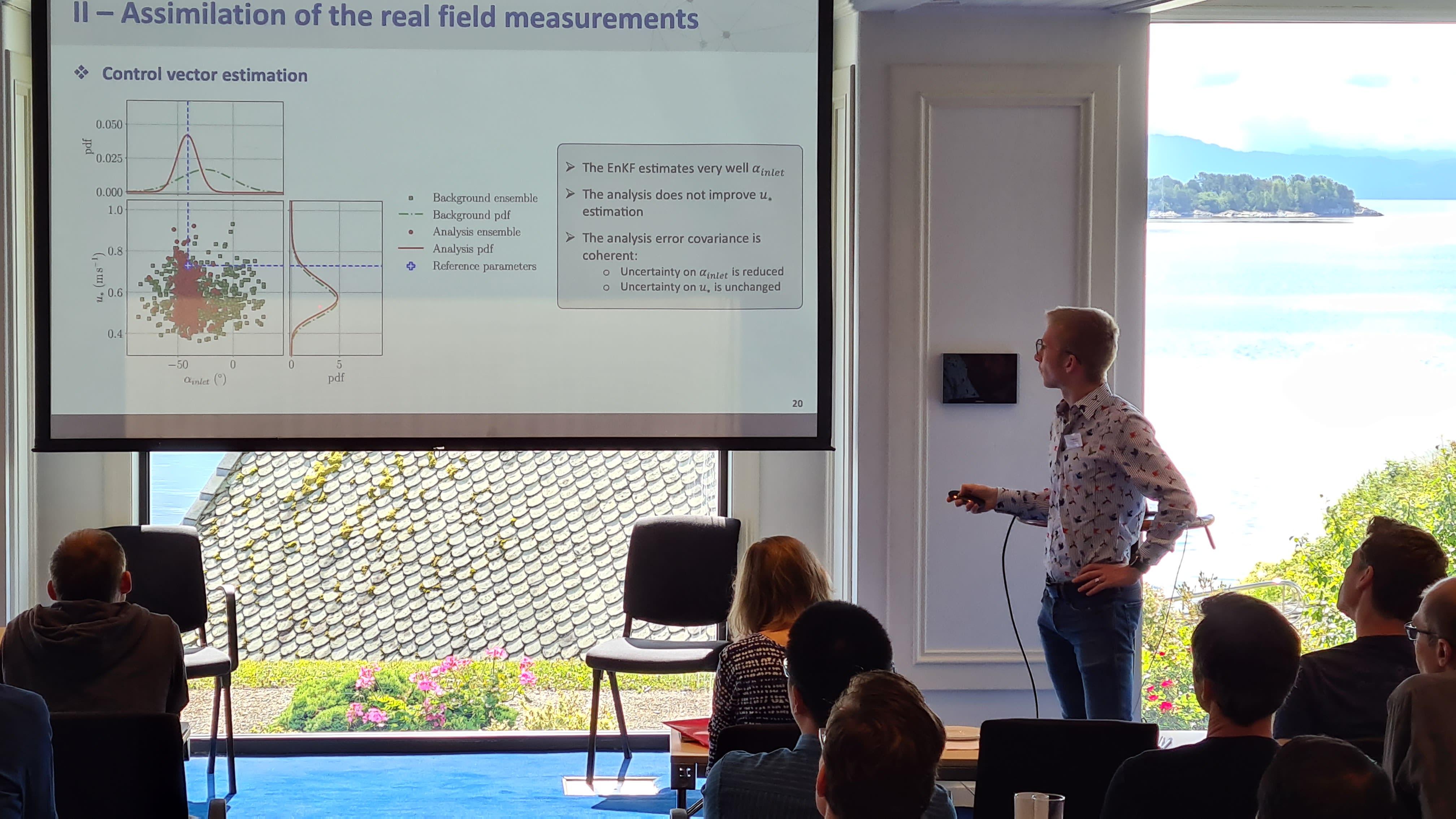
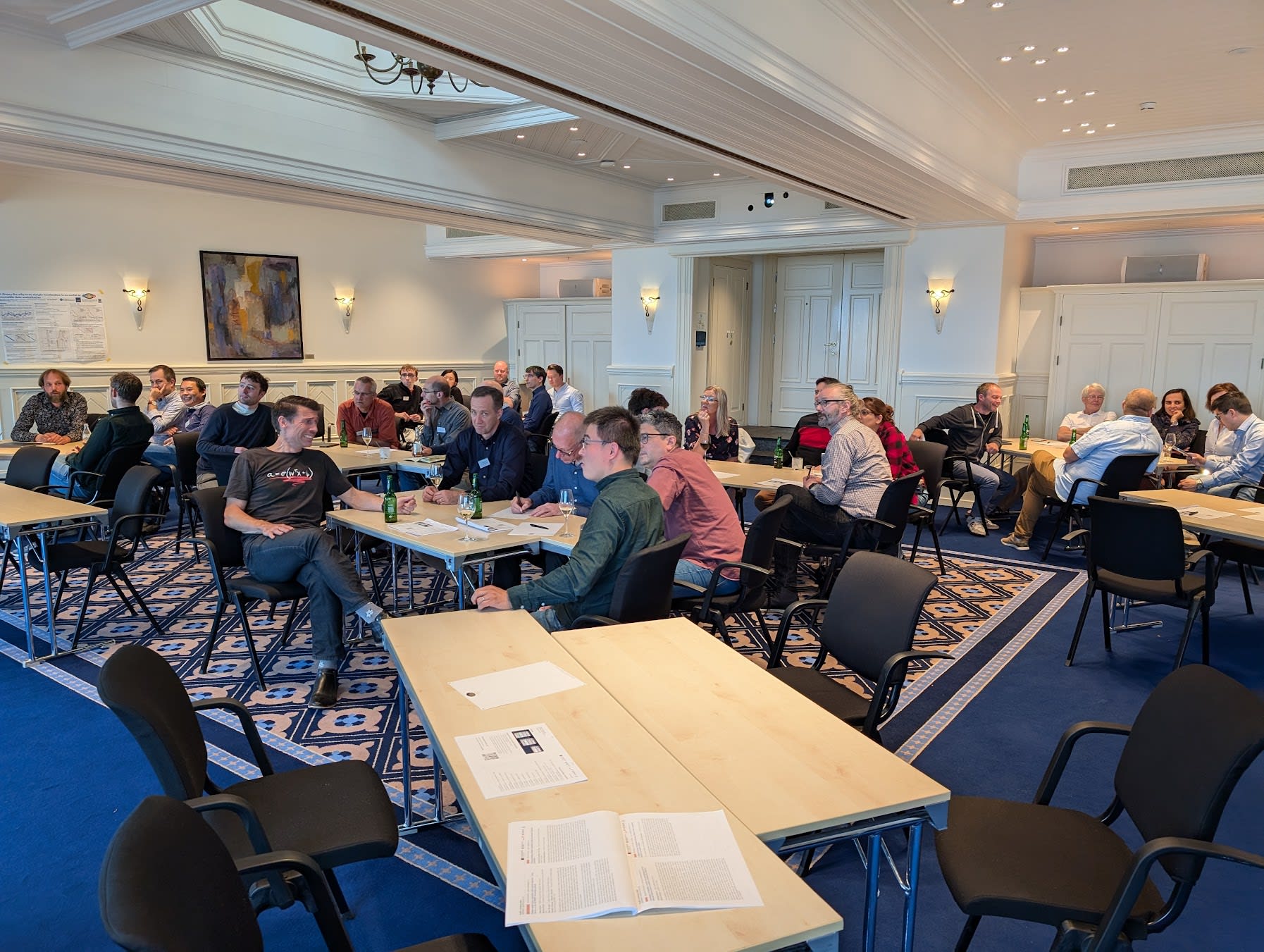
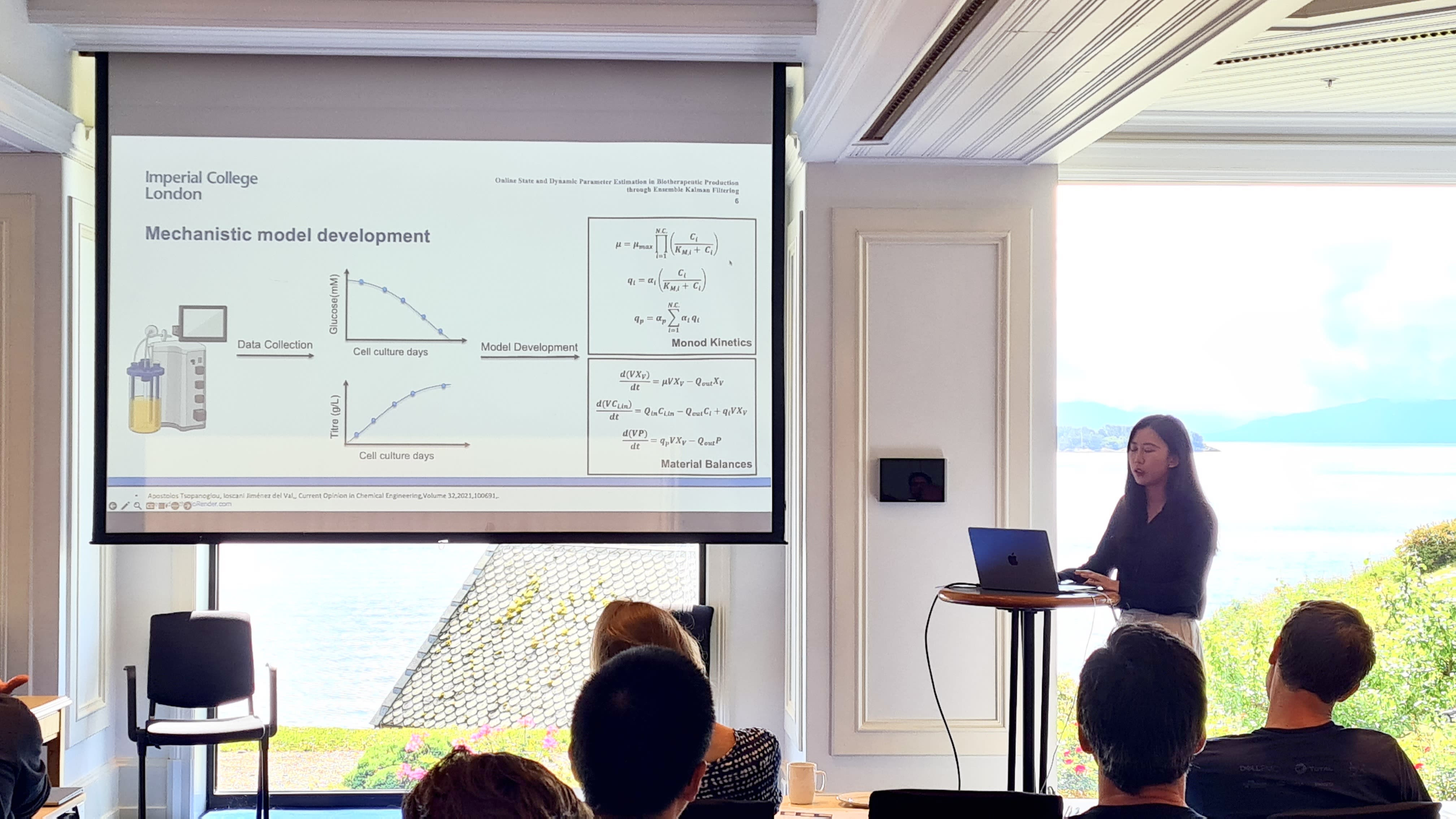
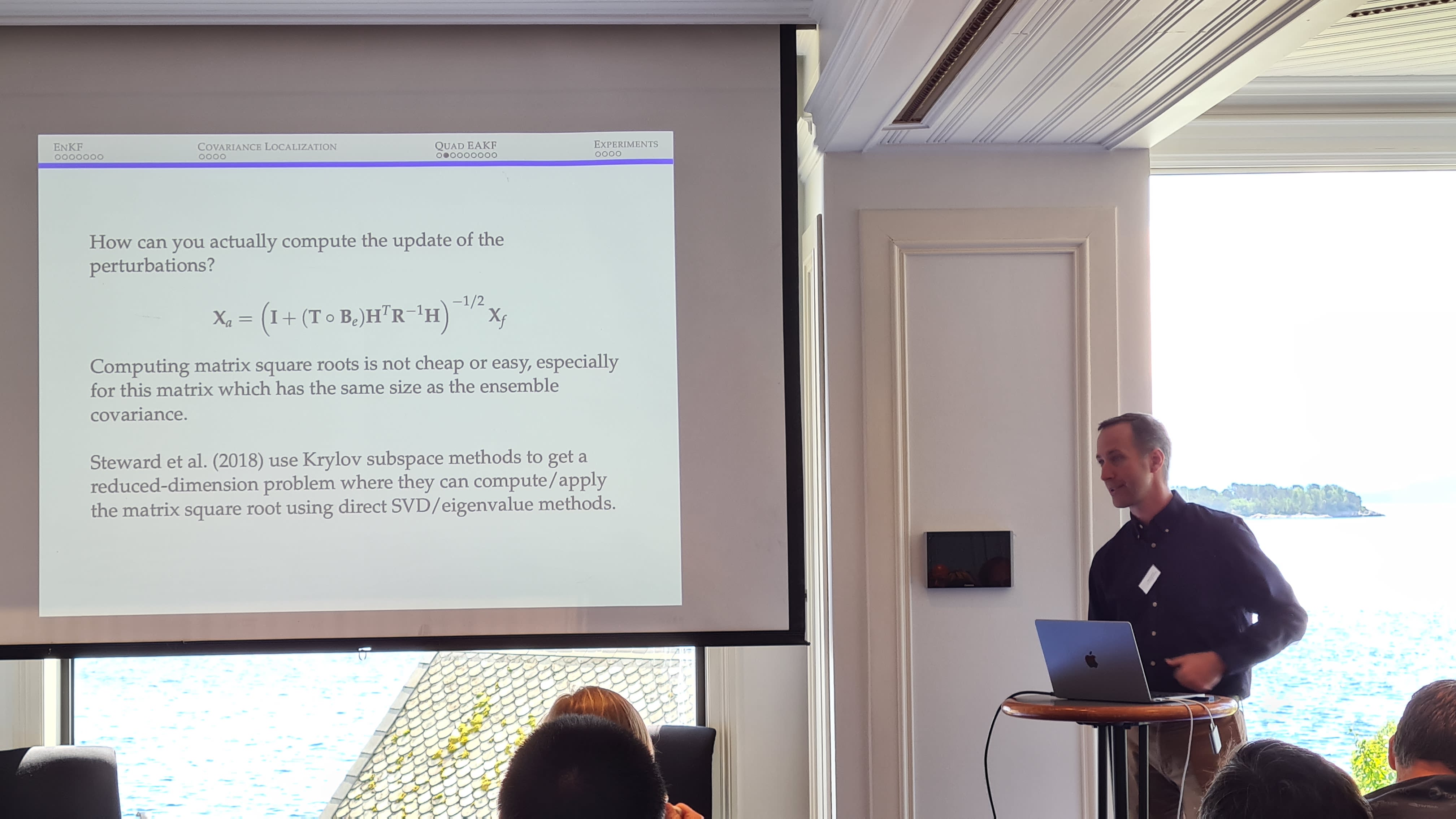
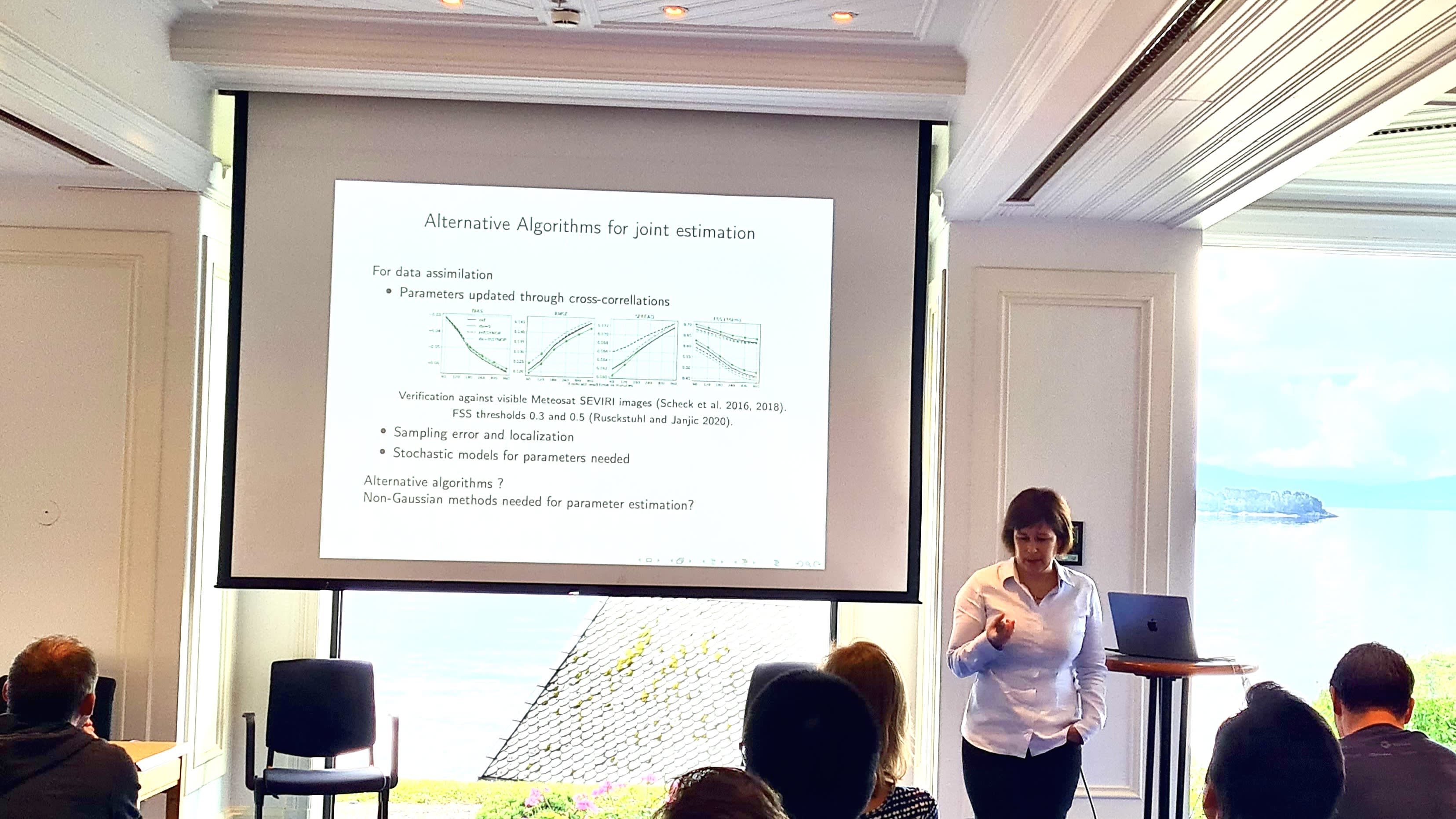
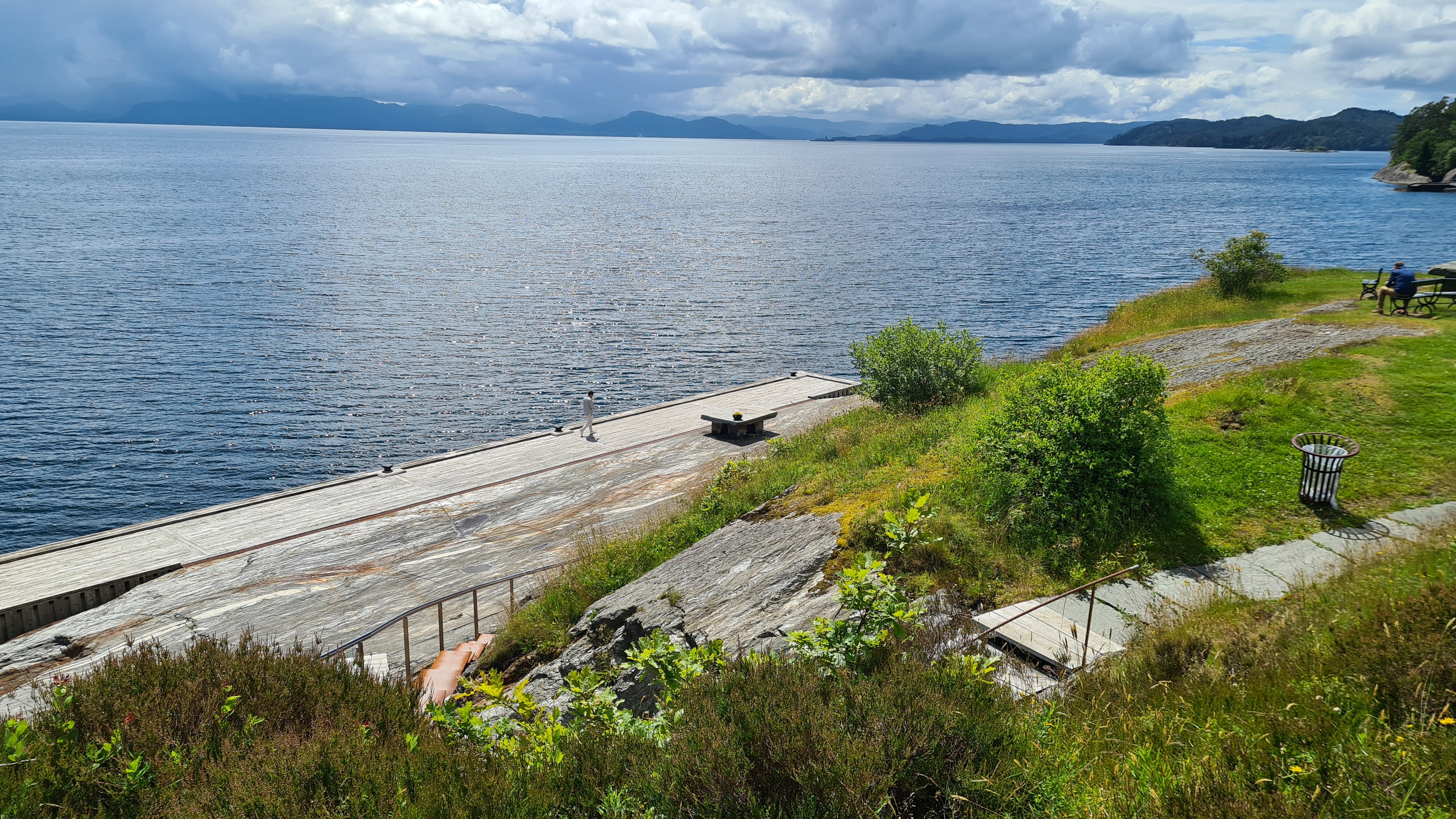
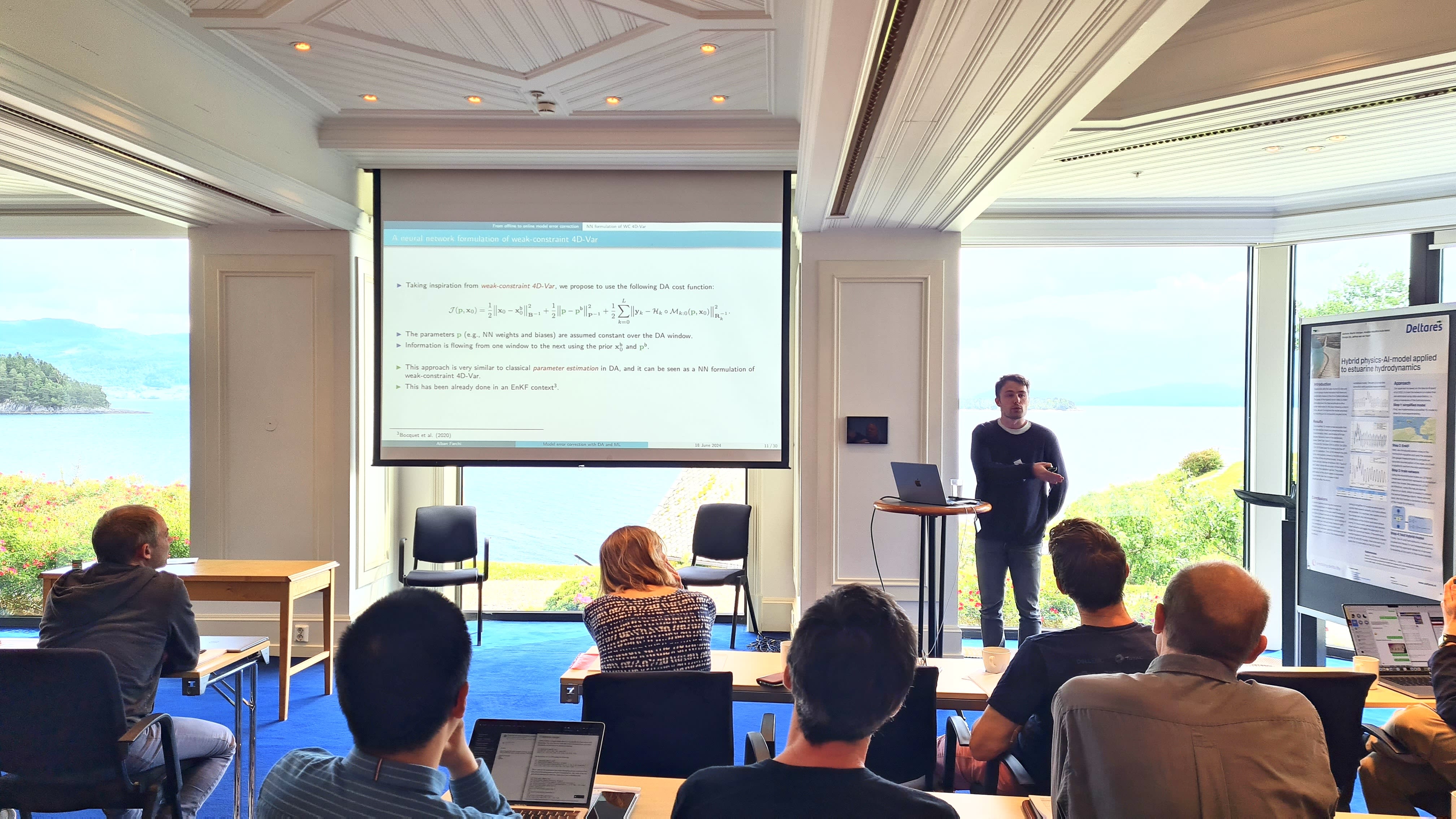
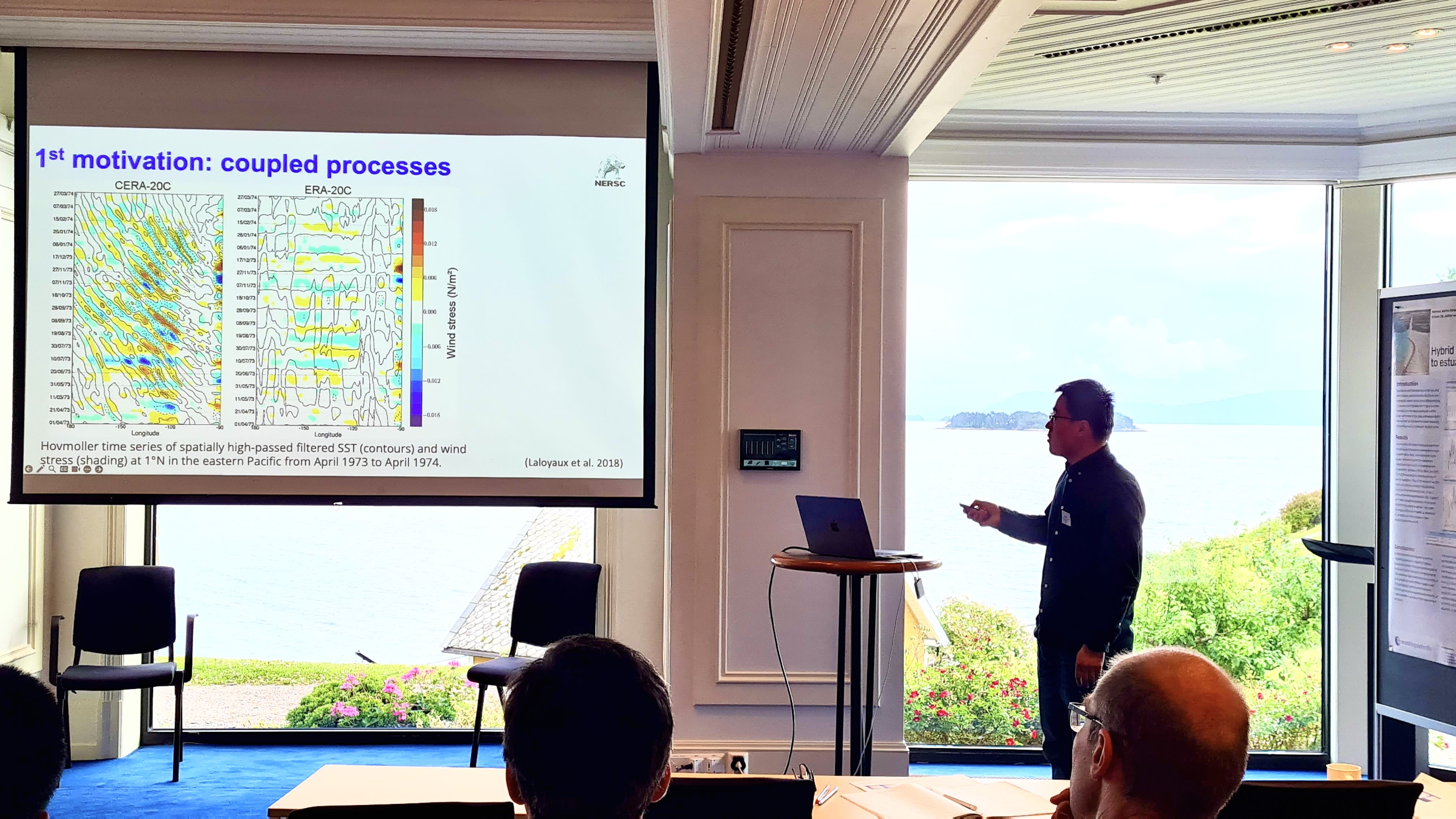
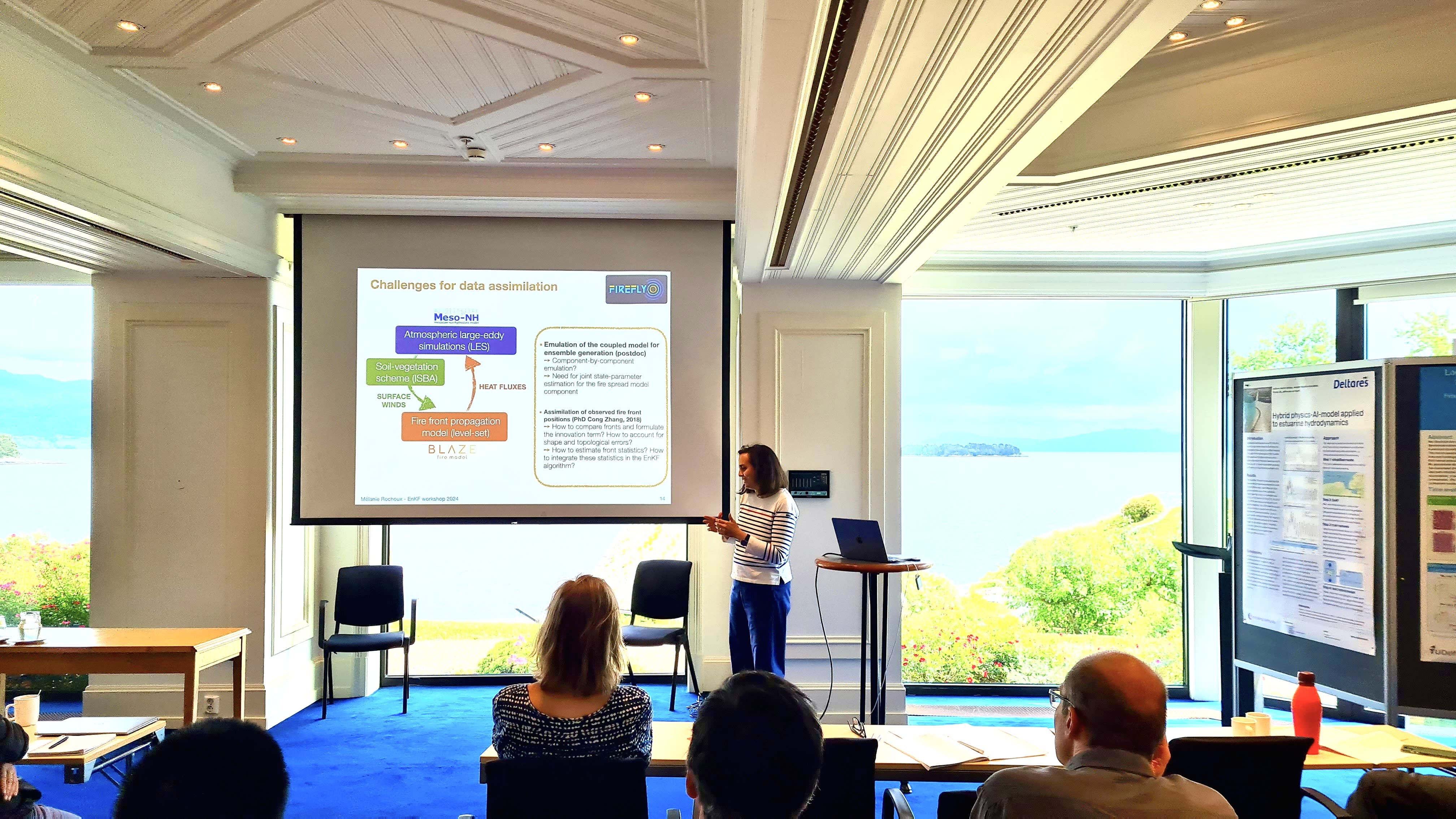
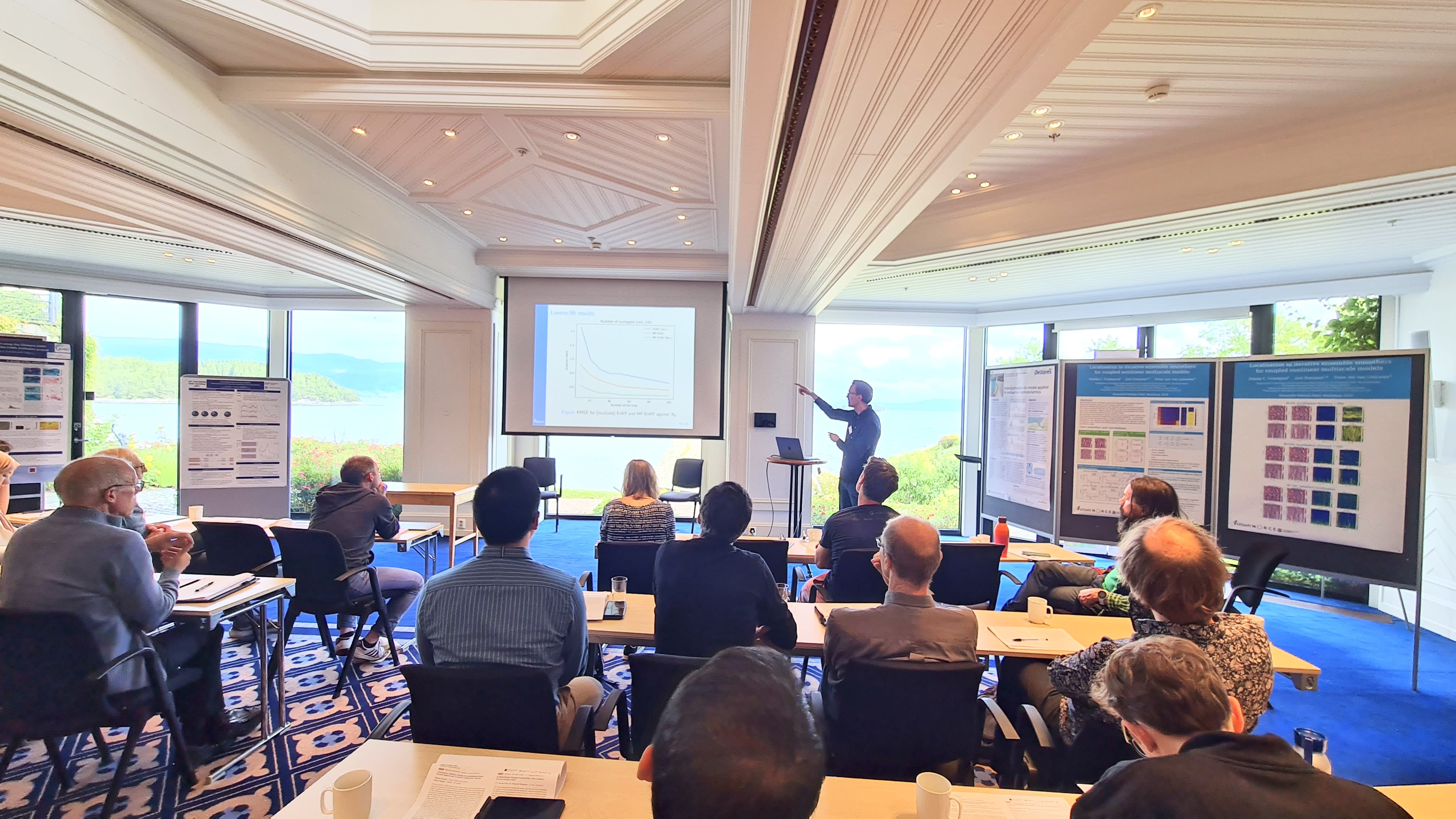
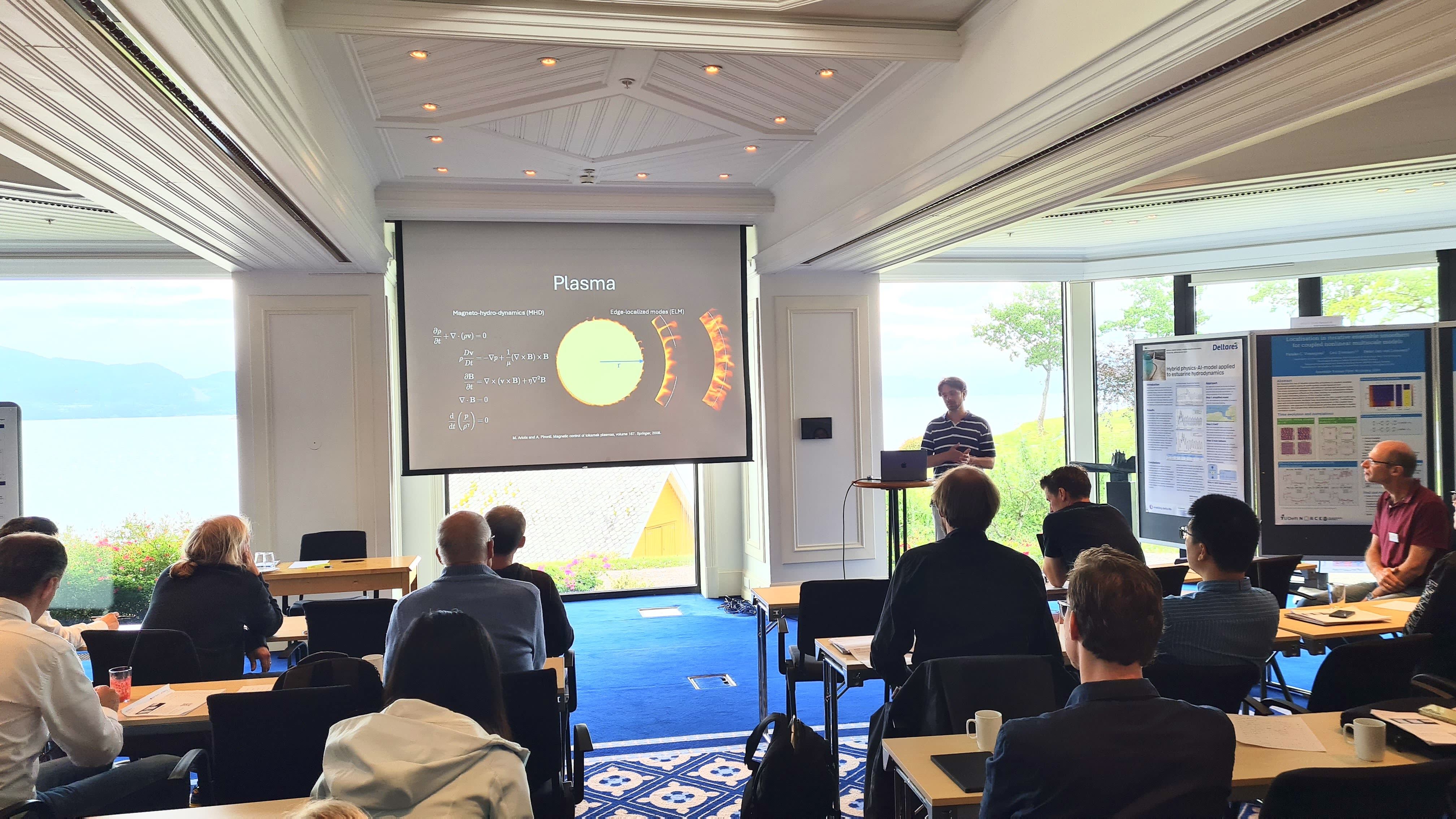
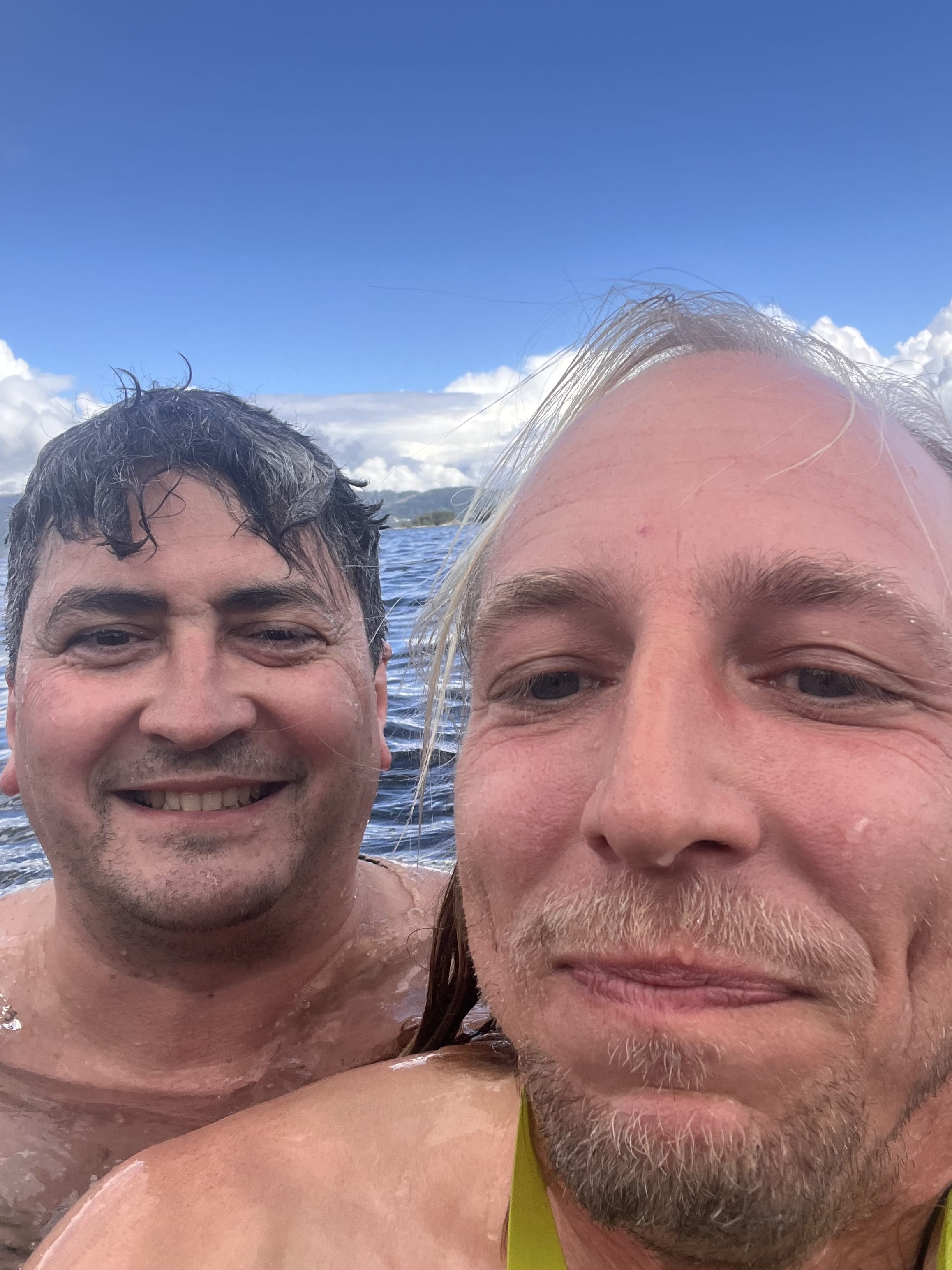
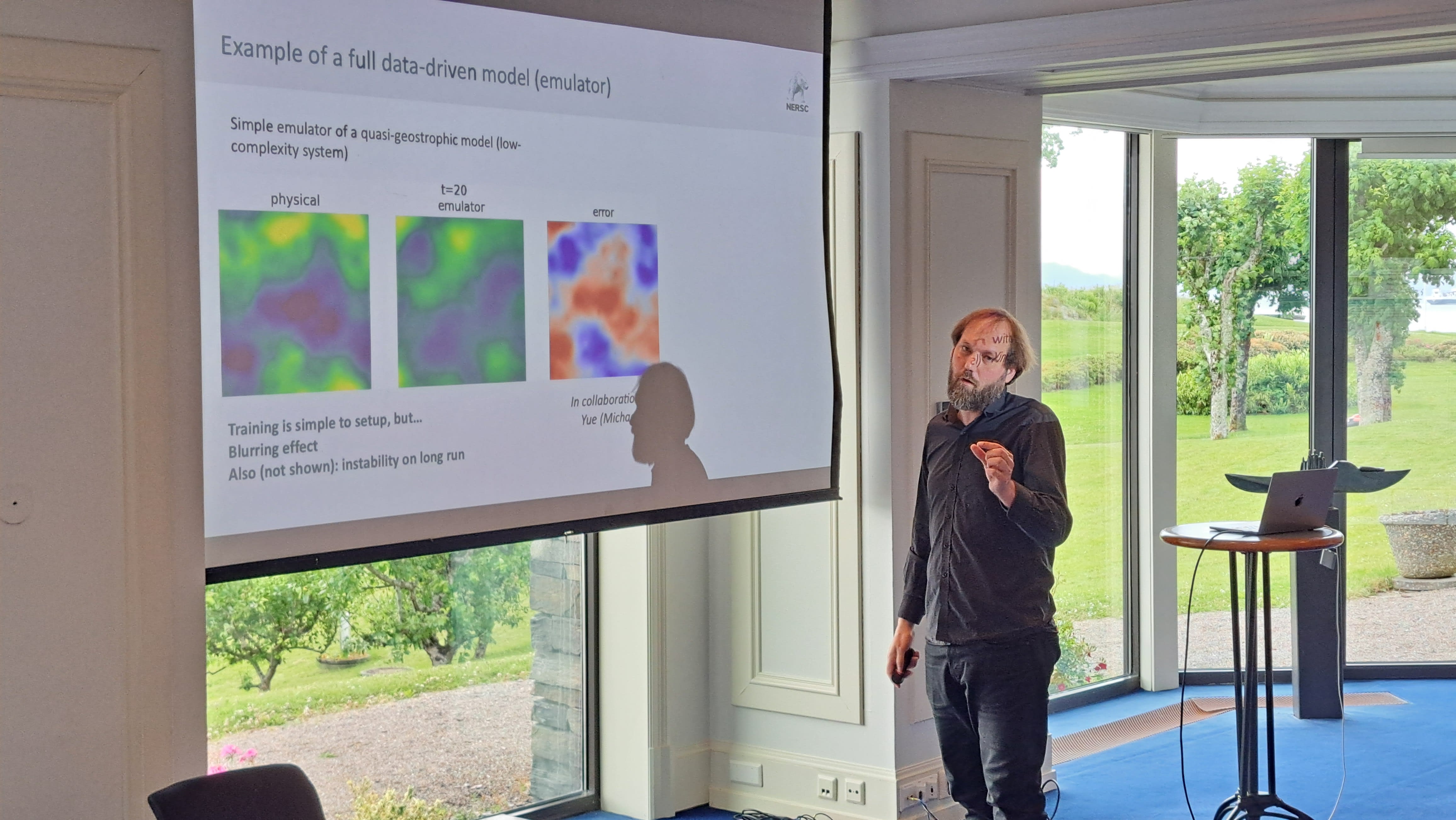
Background
The EnKF is a data assimilation method that was co-invented and has been continuously developed by researchers at NORCE and NERSC. Over the last three decades, the EnKF and related ensemble methods, has emerged as a highly effective and widely adopted approach for data assimilation in large-scale models, and have made profound impacts on the advancements of various disciplines and promoted value creations for relevant industries.
Purpose
While the fundamental concept of ensemble methods is simple, practical implementations often demand problem-specific modifications for success. Our workshop aims to bridge the expertise of specialists from various fields to investigate the foundations and interconnections of EnKF-related methods that have demonstrated effectiveness in diverse environments. In so doing, we strive to enhance the robustness and efficiency in applications. Beyond practical applications, this gathering also facilitates the exchange of innovative research ideas, methods, algorithms, and workflows with the potential to further enhance the performance of EnKF and related approaches.
History
In 2006, the inaugural EnKF workshop was held in Voss, Norway, marking the beginning of an annual tradition. Throughout the years, the EnKF workshop has consistently attracted participants from a wide range of scientific disciplines. The cross-pollination of ideas and the exchange of scientific advancements have fostered vibrant discussions and elevated the workshop’s scientific calibre to a high level. As a result, the annual international EnKF workshop has now established itself as a paramount event within the data assimilation community.
Sponsors
REMEDY
Please contact enkf@data-assimilation.no
if you want to sponsor the workshop.