14:15 at NERSC Copernicus lecture room.
Talk by Patrick Laloyaux (ECMWF),
visitor of the CoRea project.
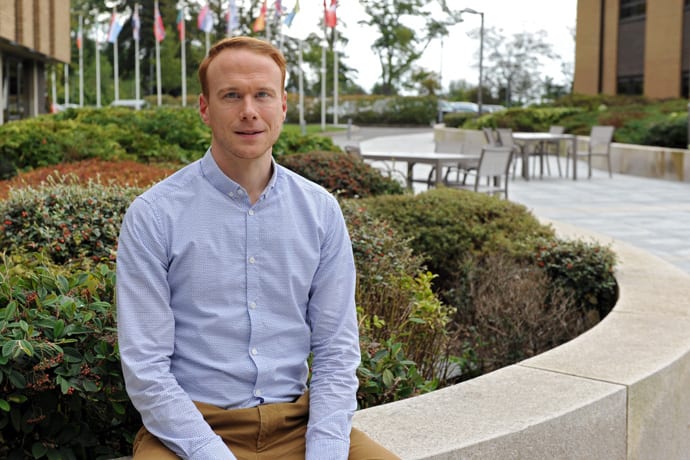
Abstract
Abstract: Recent studies have shown that it is possible to combine Machine Learning (ML) with Data Assimilation (DA) to reconstruct the dynamics of a system that is partially and imperfectly observed. This approach takes advantage of the strengths of both methods. DA is used to estimate the state of the system from the observations, while ML computes a surrogate model of the dynamical system. The surrogate model is usually defined as an hybrid combination where a physical part based on prior knowledge is enhanced with a statistical part estimated by the neural network. These developments originally aim to increase the accuracy and reliability in numerical weather prediction. We will give an overview of some recent work done at ECMWF where Neural Networks (NN) have been developed to predict the errors in the atmospheric model used in operations at ECMWF. Different approaches have been investigated to create datasets based on the information contained in analysis increments or in observations. A study of the impact of online training versus offline training is also ongoing.
The ECMWF reanalyses (most recently ERA5) are a critical resource in understanding climate change. Using a state-of-the-art data assimilation system to retrospectively analyse historical observations, we build up the best possible and most comprehensive picture of how the global atmosphere has changed since observations began up to the present day. Broadly speaking, in modern periods when we have access to huge constellations of multi-sensor carrying satellites, reanalyses are highly constrained by the observed data themselves and rely less on background information from the model. In pre-satellite times reanalyses rely much more on model information to augment the sparse observation network. Inevitably, in doing so, the resulting analyses will retain some systematic error characteristics of the model. We will present how ML solutions could alleviate this problem by computing a climatology of model error estimates (diagnosed during the present-day well observed era) to correct for model systematic error during poorly observed periods.